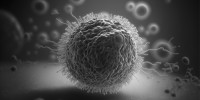
AI-Driven Model Revolutionizes Cancer Gene Prediction
A revolutionary transformer-based model is transforming cancer research by improving the identification of cancer-driver genes through graph machine learning.
At a Glance: Key Findings from the TREE Model
Transformer-Based Architecture: Uses Transformer models to analyze biological networks and multi-omics data.
Enhanced Interpretability: Provides a clearer understanding of cancer gene identification by integrating molecular features and network structure.
Identification of New Cancer Genes: Discovered 57 novel cancer gene candidates, including three not found by other models.
Multi-Omics Integration: Combines genomics, transcriptomics, and proteomics for more accurate predictions.
Network Analysis: Captures both global and local gene interactions through a co-attention mechanism.
Heterogeneous Networks: Effective in analyzing diverse molecular interactions, such as protein-protein and gene-miRNA.
Computational Efficiency: Reduces costs by training on subgraphs while maintaining high accuracy.
Real-World Applicability: Offers a generalizable approach for personalized cancer treatments across different types.
A Groundbreaking Approach to Cancer Gene Prediction: Introducing a Transformer-Based Graph Machine Learning Model
Cancer is an ever-growing global health challenge, with millions of new diagnoses and fatalities each year. The World Health Organization (WHO) reports a steady rise in cancer cases, highlighting the urgent need for innovative solutions to improve cancer prevention and treatment.
Comprehensive knowledge of human cancer genes is a critical foundation for exploring the carcinogenesis mechanism of tumour formation.![]()
Central to addressing this issue is the identification of cancer-driver genes, which play a crucial role in the development and progression of tumors. However, current methods often struggle with generalizability and interpretability, limiting their effectiveness across different cancer types and populations.
A team of researchers from the Xinjiang Institute of Physics and Chemistry at the Chinese Academy of Sciences (CAS) has proposed a new approach to overcome these challenges. Their transformer-based model, named TREE, represents a significant leap in the use of machine learning for cancer research, offering enhanced accuracy and interpretability in identifying cancer-related genes.
The TREE Framework: A Revolutionary Model for Cancer Gene Identification
TREE (Transformer-based gRaph rEpresentation lEarning) integrates graph machine learning with multi-omics data, addressing the complexities of biological networks and cancer genomics. Traditional models struggle to combine vast amounts of genomic data with the intricate molecular interactions that drive cancer. TREE, however, uses the power of the Transformer architecture—commonly employed in natural language processing (NLP)—to process graph-structured data in a way that was previously not feasible.
What sets TREE apart from conventional models is its ability to consider both the biological network's topology and multi-omics data in identifying cancer-driver genes. By incorporating structural information from biological networks, TREE not only identifies the most influential omics data but also detects the key network paths involved in regulating genes linked to cancer development.
As noted in the research published in Nature Biomedical Engineering, the model shows:
state-of-the-art performance in the prediction of cancer genes across biological networks.![]()
TREE's ability to interpret the complex relationships within these networks is particularly valuable, as it reveals the underlying regulatory mechanisms that drive cancer progression.
Key Features of TREE: How It Works
TREE’s architecture incorporates several advanced features that distinguish it from previous approaches:
Graph Structural Integration: Unlike traditional models, TREE leverages the structure of biological networks—where nodes represent biological molecules like genes, proteins, and miRNAs, and edges represent their interactions. This allows the model to capture relationships between genes and other biological factors that influence cancer development.
Multi-Omics Data Integration: TREE combines data from various omics sources, such as genomics, transcriptomics, and proteomics, to identify cancer-driver genes more effectively. The model incorporates both the gene's molecular features and the broader context of its interactions in the biological network.
Co-Attention Mechanism: One of TREE's standout features is its co-attention mechanism, which enhances the model’s ability to capture complex global relationships between genes. This mechanism integrates global structural information with node-level attention, guiding the model’s focus on the most important gene interactions.
Heterogeneous Network Handling: TREE excels in working with heterogeneous networks, which include different types of molecular interactions (such as protein-protein interactions, gene-miRNA interactions, and transcription factor-gene interactions). This capability is crucial for capturing the full spectrum of regulatory mechanisms in cancer biology.
Efficient Computational Resources: Despite the complexity of its design, TREE efficiently learns from subgraphs sampled from the larger network. This reduces computational costs while maintaining high performance, making it feasible for large-scale applications.
Major Achievements: Unveiling New Cancer Genes
The TREE model has shown impressive results in predicting cancer genes. In one of its key findings, TREE successfully identified 57 novel cancer-gene candidates from a pool of 4,729 unlabelled genes across eight pan-cancer datasets. Among these, three genes had not been identified by previous models, demonstrating the model’s ability to uncover previously overlooked targets.
The model also highlights the importance of specific omics data types, such as genetic mutations, in cancer gene prediction. According to the research, "mutations primarily contribute to the identification of cancer genes," underscoring the significance of genomic alterations in cancer formation.
Moreover, TREE’s ability to interpret network patterns offers new insights into the regulatory mechanisms of cancer genes. For instance, the gene–gene–transcription factor–gene metapath was found to be particularly informative in identifying cancer-driving genes.
Transforming Cancer Research and Treatment
The potential impact of TREE extends far beyond cancer research. By enhancing the interpretability and generalizability of cancer gene prediction, TREE can help researchers and clinicians better understand the molecular mechanisms underlying cancer. This knowledge can ultimately lead to more precise, personalized treatment strategies tailored to an individual’s unique genetic makeup and cancer profile.
TREE’s approach is also highly adaptable, with the potential to be applied to other diseases and biological networks, making it a versatile tool for a wide range of biomedical research applications.
Looking Ahead
The development of TREE marks an important step forward in the integration of artificial intelligence with biomedical research. By leveraging cutting-edge machine learning techniques, TREE offers a powerful tool for deciphering the complex networks of interactions that drive cancer. As cancer genomics continues to evolve, TREE’s ability to uncover new insights into gene regulation and cancer progression promises to play a key role in the ongoing fight against this global health threat.
With its robust performance and interpretability, TREE holds the potential to revolutionize not just cancer research, but also the broader field of precision medicine. As stated in the study:
The model’s interpretability and generalization may facilitate the understanding of gene-related regulatory mechanisms and the discovery of new cancer genes![]()
– paving the way for more effective treatments and, ultimately, better patient outcomes.