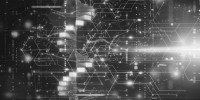
AI-Powered OmicsFootPrint Transforms Disease Research
AI is transforming biomedical research, and OmicsFootPrint is at the forefront. This AI-powered tool from Mayo Clinic turns complex biological data into intuitive circular maps, enhancing disease classification and drug response predictions.
OmicsFootPrint: AI-Powered Insights into Disease Mechanisms
The integration of artificial intelligence (AI) with biomedical research continues to transform the understanding of complex diseases. Researchers at Mayo Clinic have introduced OmicsFootPrint, an AI-driven tool designed to interpret multi-omics data through intuitive circular images.
This innovative approach enhances disease classification, drug response prediction, and the discovery of new therapeutic targets. Published in Nucleic Acids Research, the study highlights the potential of OmicsFootPrint in advancing precision medicine.
At a Glance
AI-powered visualization: converts complex multi-omics data into intuitive circular maps.
High accuracy: achieves over 95% accuracy in identifying lung cancer subtypes.
Multi-omics integration: improves disease classification by combining genetic, proteomic, and molecular data.
Efficient data processing: reduces data storage needs to just 2% of the original size.
Clinical and research applications: Supports disease mechanism exploration and potential integration into electronic health records.
A New Era in Disease Mapping
OmicsFootPrint leverages deep neural networks to process vast amounts of biological data, helping researchers uncover patterns in diseases such as cancer and neurological disorders. By translating complex genetic and molecular interactions into visually interpretable circular images, the tool allows for a more intuitive exploration of disease mechanisms.
The framework was tested using The Cancer Genome Atlas (TCGA) dataset, where it distinguished between invasive lobular and ductal breast carcinomas with 87% accuracy. For lung cancer, it achieved an impressive 98% accuracy (AUC: 0.98 ± 0.02) in differentiating adenocarcinoma from squamous cell carcinoma. Furthermore, in drug response predictions, OmicsFootPrint outperformed nine existing methods, demonstrating a median AUC of 0.74 in predicting drug efficacy in cancer cell lines.
Enhancing Interpretability with SHAP
One of the key features of OmicsFootPrint is its incorporation of SHapley Additive exPlanations (SHAP), an advanced AI method that enhances model interpretability. SHAP identifies the most critical markers—such as specific genes and proteins—that contribute to disease classification. This allows researchers to pinpoint key drivers of disease progression, aiding in the development of targeted treatments.
Data becomes most powerful when you can see the story it's telling.![]()
By visualizing disease-related molecular changes, OmicsFootPrint provides a new way to explore complex interactions in disease pathology.
From Research to Clinical Application
Beyond research, OmicsFootPrint has the potential to be integrated into electronic medical records (EMRs). The tool compresses large datasets into compact images, facilitating seamless storage and retrieval. This capability could revolutionize clinical decision-making, allowing healthcare providers to access critical molecular insights in real time.
The research team is now working on expanding the application of OmicsFootPrint to neurological and other complex diseases. Future updates aim to further refine accuracy, enhance flexibility, and uncover novel biomarkers and drug targets.
The Future of AI in Precision Medicine
The OmicsFootPrint framework addresses the need for advanced multi-omics data analysis methodologies by transforming data into intuitive two-dimensional circular images and facilitating the interpretation of complex diseases.![]()
Utilizing deep neural networks and incorporating the SHapley Additive exPlanations algorithm, the framework enhances model interpretability.
OmicsFootPrint represents a significant advancement in multi-omics research, bridging the gap between vast biological datasets and actionable clinical insights. By integrating AI-driven analysis with intuitive visualization, this tool holds promise for revolutionizing disease diagnosis, treatment, and precision medicine.
As AI continues to evolve, tools like OmicsFootPrint will play a critical role in shaping the future of biomedical research, offering faster, more accurate, and highly interpretable solutions for complex disease analysis.