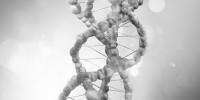
Deep Learning Solutions for Omics Data Challenges
Omics technologies generate vast amounts of complex data, but deep learning offers powerful solutions to unlock their full potential. Discover how AI can overcome key challenges in omics research, from model interpretability to ethical compliance.
Harnessing Deep Learning to Tackle Challenges in Omics Data Analysis
Omics technologies have transformed biological research, providing in-depth insights into genomics, transcriptomics, proteomics, and metabolomics. These advancements have significantly contributed to precision medicine and disease research. However, omics data analysis presents significant challenges due to its complexity and vast scale.
Deep learning (DL) has emerged as a powerful tool to unlock patterns within omics data. However, despite its potential, challenges such as model interpretability, data scarcity, and computational costs remain. This article explores these challenges and the strategies researchers can employ to overcome them.
The Complexity and Scale of Omics Data
Omics research generates massive datasets through high-throughput sequencing and mass spectrometry. The sheer volume of this data makes storage, management, and analysis a formidable task. Traditional statistical methods often struggle with high-dimensional, unstructured data, limiting the insights researchers can extract.
Deep learning models have shown promise in handling such complexity by identifying patterns that traditional methods might miss. However, their implementation comes with obstacles that must be addressed for successful integration into biomedical research.
Overcoming Challenges with Deep Learning
1. Enhancing Model Interpretability
One of the key barriers to deep learning adoption in omics research is the lack of interpretability. Clinicians and researchers must understand how models make predictions to ensure trust and reliability.
Solutions:
Explainable AI (XAI): Techniques like SHAP (SHapley Additive exPlanations) and Grad-CAM can provide insights into how DL models arrive at their conclusions.
Attention Mechanisms: These can highlight critical features in omics datasets, making it easier to interpret results.
2. Addressing Data Scarcity
Deep learning models require large datasets to perform effectively, yet omics datasets are often limited due to cost and ethical concerns.
Solutions:
Transfer Learning: Using pre-trained models on related datasets can help DL models learn effectively with limited data.
Data Augmentation: Techniques like synthetic data generation and oversampling can improve model performance when real data is scarce.
3. Reducing Computational Costs
Deep learning models, especially deep neural networks, require significant computational power, which can be a barrier to widespread adoption.
Solutions:
Optimized DL Architectures: Streamlining network structures can reduce computational demands without sacrificing performance.
Hardware Accelerators: Leveraging GPUs and TPUs can improve model efficiency and reduce processing time.
Ensuring Ethical Compliance in Omics Research
With the increasing reliance on deep learning, it is crucial to ensure data privacy and ethical compliance. Anonymization of patient data and adherence to ethical guidelines are essential to maintaining trust in biomedical research.
Conclusion
Despite the challenges associated with omics data analysis, deep learning presents exciting opportunities for uncovering new biological insights. By improving model interpretability, addressing data limitations, and optimizing computational efficiency, researchers can fully leverage the power of DL in omics research.
As the field evolves, interdisciplinary collaboration and ethical considerations will be crucial in unlocking the full potential of omics technologies and driving advancements in precision medicine.