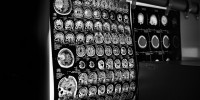
How AI and Imaging Are Revolutionizing MS Diagnosis
Discover how cutting-edge AI and imaging technologies are revolutionizing multiple sclerosis diagnosis, achieving remarkable accuracy and reliability. Dive into the future of medical diagnostics!
AI and Imaging Advances Revolutionize MS Diagnosis
In recent years, advancements in imaging technology and artificial intelligence (AI), particularly machine learning (ML), have dramatically improved the diagnosis and classification of multiple sclerosis (MS). A new study published in Translational Vision Science & Technology highlights the potential of using infrared reflectance scanning laser ophthalmoscopy (IR-SLO) images alongside optical coherence tomography (OCT) to automate MS diagnosis.
Incorporating both modalities improves the performance of automated diagnosis of MS, showcasing the potential of utilizing IR-SLO as a complementary tool alongside OCT.![]()
At a Glance:
Disease: Multiple Sclerosis (MS)
Tech Used: Optical Coherence Tomography (OCT), Infrared Reflectance Scanning Laser Ophthalmoscopy (IR-SLO), Machine Learning (ML)
Study Results:
Accuracy: 92%
Sensitivity: 95%
Specificity: 92%
Discriminative Ability (AUROC curve): 97%
Automating the Diagnosis of MS
Multiple sclerosis is an autoimmune disorder that causes demyelination and breakdown of the central nervous system (CNS) and neural axons. Traditionally, MS diagnosis relies on clinical symptoms, laboratory tests, and magnetic resonance imaging (MRI). However, recent studies show that OCT can detect early changes in the retinal nerve fibers, often correlating with MRI findings, MS subtypes, and patient disabilities.
The Role of OCT and AI
OCT measures the thickness of retinal layers, which can indicate the progress of neurodegeneration. AI and ML have been integrated with OCT, MRI, serum, and cerebrospinal fluid (CSF) data to automate MS diagnosis. However, the independent diagnostic capabilities of IR-SLO, a technology often used in conjunction with OCT, had not been fully explored—until now.
About the Study
In the study, both OCT and IR-SLO images were analyzed from 32 MS patients and 70 healthy controls. Researchers used multiple convolutional neural networks (CNNs) to train models on these datasets. The combined model using both OCT and IR-SLO data achieved superior performance compared to models using either technology alone.
Sample Size:
Healthy Controls: 70 individuals
MS Patients: 32 individuals
Data:
IR-SLO Images: 265
OCT Images: 184
Study Results
The combined model demonstrated:
Accuracy: 92%
Sensitivity: 95%
Specificity: 92%
Area Under the Receiver Operating Characteristic (AUROC) Curve: 97%
Area Under the Precision-Recall Curve (AUPRC): 97%
A quote from the study summarizes its groundbreaking nature:
To our knowledge, this is a pioneering study incorporating IR-SLO into automated diagnosis of MS … among the few studies that have applied DL to retinal imaging data for detecting MS.![]()
Conclusions and Future Directions
While models trained only with IR-SLO data did not perform as well as those using OCT thickness data, the combined approach resulted in a 3% increase in accuracy and sensitivity. This indicates that using both imaging modalities allows for a more comprehensive extraction of features necessary for MS diagnosis.
The study showcases how AI-based systems can identify minor changes in the optic nerve that may escape human observation. Such advancements could lead to less invasive, more cost-effective, and routine clinical practices for diagnosing MS.
Future research should focus on larger, more diverse validation studies to further enhance the reliability and applicability of these findings. The integration of these AI-driven diagnostic tools in clinical settings could revolutionize how MS is diagnosed and monitored, offering hope for better patient outcomes.
For those interested in the technical details and further reading, the original study can be accessed through Translational Vision Science & Technology.