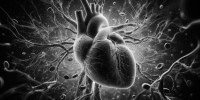
Machine Learning and the Exposome: Unlocking CVD Secrets
Discover how machine learning is transforming our understanding of cardiovascular diseases by analyzing the complex interplay of environmental and lifestyle factors, collectively known as the "Exposome."
Exploring the Role of Machine Learning in Understanding Exposomics and Cardiovascular Diseases
A new article by researchers from Greece highlights recent advances and challenges in this emerging field and offers promising insights into personalized healthcare and public health strategies. Dive in and explore the future of cardiovascular disease prevention and management.
At a Glance:
This article presents findings from a new study on machine learning applications in exposomics and cardiovascular diseases.
Cardiovascular diseases (CVDs) are the leading cause of death globally.
Environmental and lifestyle factors, termed as the "Exposome," significantly influence CVD risk.
Machine learning (ML) is emerging as a powerful tool to analyze complex exposomic data.
Current research is predominantly conducted in the US and Asia, with Europe showing increasing interest.
The field is rapidly expanding, especially since 2021.
Introduction
Cardiovascular diseases (CVDs) have long held the grim title of the world’s leading cause of death, claiming over 20 million lives annually. In Europe alone, CVDs are responsible for 3.9 million deaths each year, imposing a staggering financial burden of 210 billion euros on the EU economy. While traditional risk factors like blood pressure and cholesterol have been targeted for prevention, the complex interplay of environmental and behavioral factors, collectively known as the "Exposome," has garnered growing attention as a critical area for research and intervention.
Understanding the Exposome
The term "Exposome" was first introduced by Dr. Christopher Wild in 2005 to complement genetic research and provide a holistic view of environmental exposures affecting health from conception onwards. It encompasses a wide range of factors such as diet, air quality, social interactions, and lifestyle choices like smoking and exercise. By measuring these exposures, researchers aim to pinpoint modifiable risk factors and devise targeted interventions to promote cardiovascular health.
Machine Learning: A New Hope for CVD Research
Traditional epidemiological studies often struggle with the highly heterogeneous and dynamic nature of exposomic data. This is where machine learning (ML) steps in. ML techniques, including deep learning (DL), are particularly well-suited to handle large, complex datasets, uncover intricate relationships, and enhance causal inference capabilities. Despite being relatively new to the field of exposomics, ML is increasingly adopted to investigate various aspects of cardiovascular diseases.
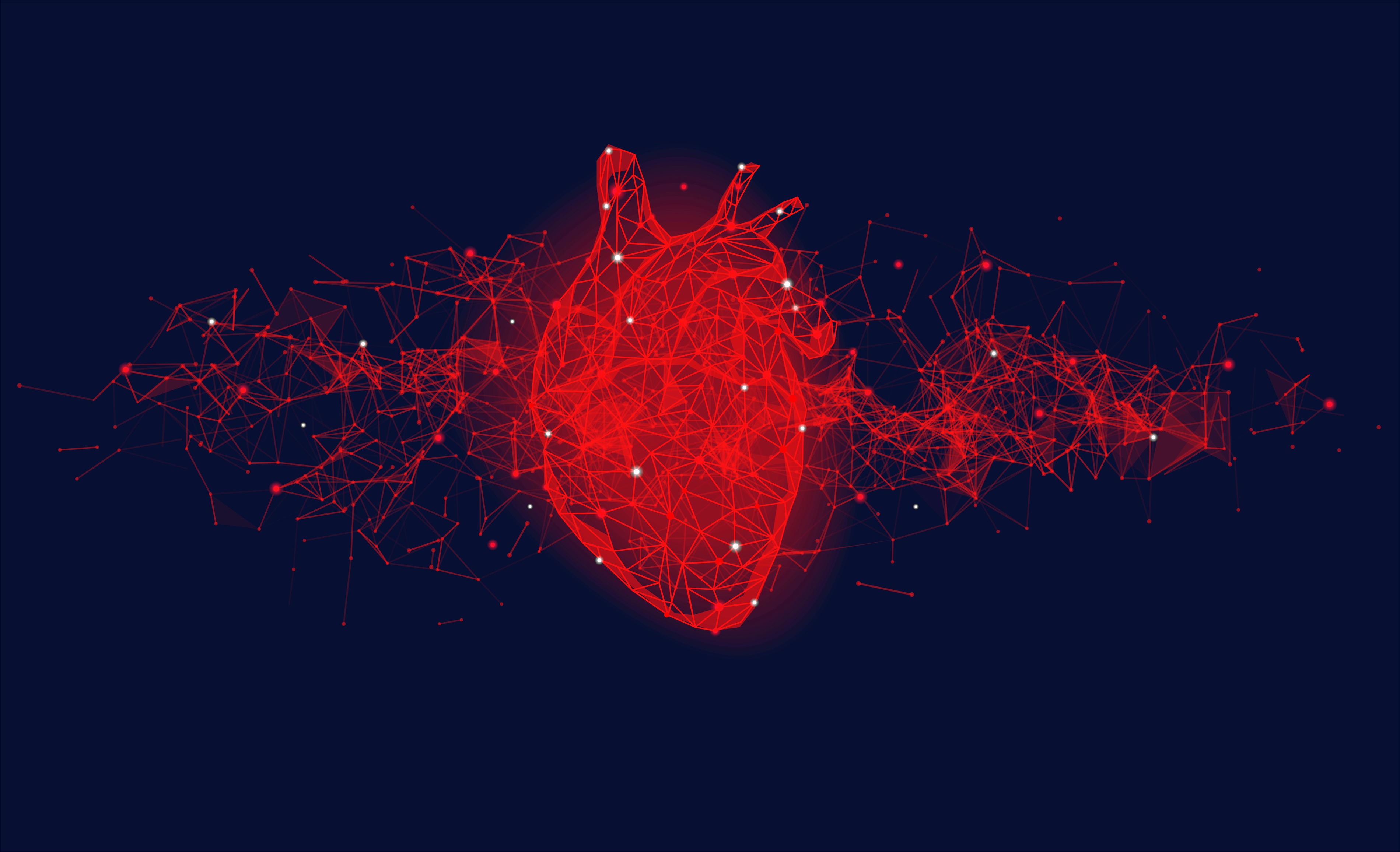
Key Areas of Research in ML and Exposomics
Forecasting Cardiovascular Outcomes
One major category of studies focuses on predicting cardiovascular outcomes such as disease incidence, severity, and mortality. For instance, Guimbaud et al. developed early-life environmental risk scores to assess the impact of environmental exposures on health outcomes, including cardiometabolic health. Other studies aim to identify at-risk neighborhoods or individuals, thereby contributing to more informed public health strategies.
Predicting Healthcare Resource Demand
Another significant category targets healthcare resource management by forecasting variables like the number of CVD-induced hospital admissions. For example, Lin et al. developed ML models to predict emergency department visits related to CVD, aiming to optimize clinical manpower and resource allocation.
Popular Machine Learning Techniques
Ensemble algorithms, particularly Random Forest and XGBoost, are the most widely adopted ML methods in this field. These techniques are often compared against linear and non-linear algorithms, with ensemble methods frequently emerging as top performers. However, there's ample room for exploring under-researched unsupervised techniques to enhance predictive modeling further.
Key Exposomic Factors Investigated
Environmental exposure is the most widely studied category within the exposome, including factors like air pollutant concentrations, noise levels, and meteorological parameters. Socio-economic factors such as income, education, and employment status also play a significant role. Lifestyle-related factors, primarily dietary habits, physical activity, and smoking status, are frequently addressed in combination with environmental and socio-economic factors.
Challenges and Opportunities
Despite promising advancements, several limitations persist. The validity of ground truth data, subjectivity in self-reported information, and regional biases in data collection are notable challenges. There's also a lack of standardization in terms and definitions, making it difficult to compare results across studies.
However, the growing interest and investment in exposomics research, particularly in the EU, offer a bright outlook. As ML applications in this field mature, they hold the promise of uncovering new insights into the environmental determinants of health and identifying valuable strategies for CVD prevention and healthcare resource allocation.
Conclusion
Machine learning techniques applied to exposomics data are still in their nascent stages compared to other -omics fields like genomics. However, the rapid increase in relevant publications, particularly from 2021 onwards, highlights the growing interest and potential in this area. As the research community continues to explore the modifiable factors within the exposome, we move closer to developing personalized healthcare management tools and optimizing public health strategies. As the study notes:
The fact that environmental CV risks factors are inherently preventable leads to the actionable conclusion that reducing them is a key-step to alleviating the burden of cardiovascular disease in Europe.![]()
The journey towards understanding the exposome and its impact on cardiovascular diseases is just beginning. With continued research, collaboration, and standardization, we can look forward to more effective interventions and a healthier future.
By integrating machine learning with exposomics, we stand on the brink of groundbreaking discoveries that could revolutionize cardiovascular disease prevention and management. Stay tuned for more updates and insights as this exciting field continues to evolve.