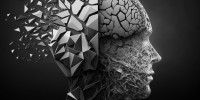
Machine Learning Enhances Multidomain Dementia Prediction
Discover how a recent study leverages machine learning to enhance dementia prediction by integrating multiple risk factors, from clinical history to neuroimaging biomarkers. Learn which predictors offer the most value and how this innovative approach could revolutionize early detection and intervention. Let's go!
Unlocking the Secrets to Dementia Prediction: A Study on Multidomain Risk Factors
Dementia is a complex and multifactorial neurodegenerative condition that affects millions worldwide. As the population ages, the need for accurate and early prediction of dementia becomes increasingly critical. A recent study delves into the various domains that contribute to the prediction of dementia, including Alzheimer's disease (AD) and vascular dementia (VaD).
Let's explore the key findings and implications of this study!
At a Glance
Study Objective: Assess the incremental value of five predictor domains (clinical and medical history, questionnaire, cognition, polygenetic risk, and neuroimaging biomarkers) for predicting dementia.
Main Finding: Neuroimaging biomarkers showed the highest incremental value for predicting dementia and AD. Clinical and medical history data were best for predicting VaD.
Implications: The findings can help practitioners select the most relevant predictors for developing machine learning (ML)-based dementia risk prediction models.
Breaking Down the Research
Study Design and Participants
The study was based on data from the UK Biobank, a large population-based cohort study. It included individuals aged 60 or older without dementia at baseline.
Various data were collected, including clinical and medical history, questionnaire responses, cognitive test results, polygenetic risk scores, and neuroimaging biomarkers.
Key Findings
Neuroimaging Biomarkers: The Game Changer
The study found that neuroimaging biomarkers provided the highest incremental value when added to a simple dementia risk prediction model (DRPM).
Specifically, these markers improved the model's Area Under the Curve (AUC) by 9.6% for all-cause dementia and 16.5% for AD. This suggests that brain imaging data can significantly enhance the accuracy of predicting dementia.
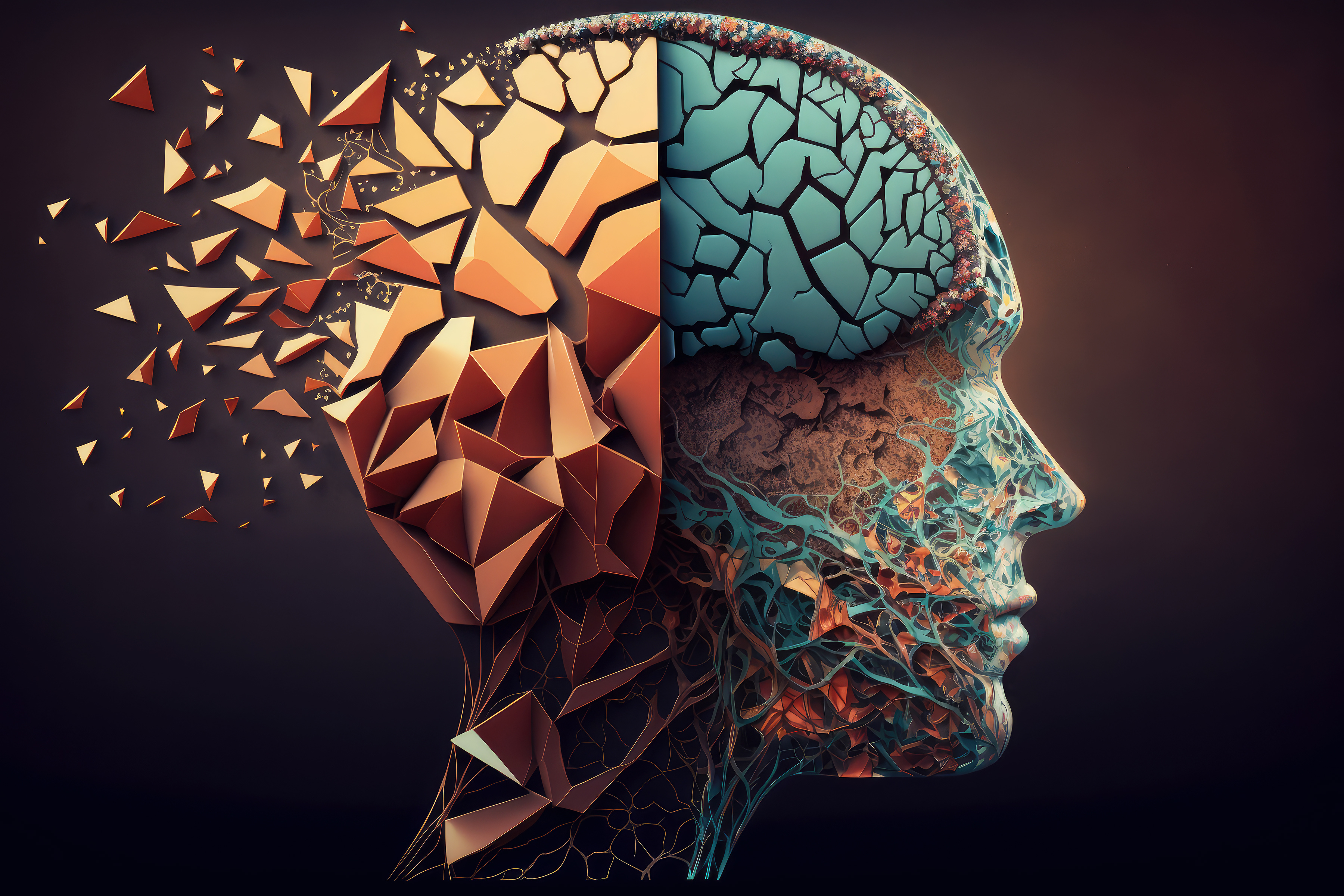
Clinical and Medical History: Best for VaD
For predicting vascular dementia, clinical and medical history data were the most effective, adding 12.2% to the model's AUC. This domain includes factors like blood pressure, cholesterol levels, and history of heart problems, which are well-known risk factors for VaD.
Combining Domains: Synergistic Effects
Combining predictors from different domains generally resulted in better predictive performance. For example, integrating clinical and medical history with questionnaire data improved the AUC by 12.1%. Adding advanced predictors like polygenetic risk scores or neuroimaging biomarkers further enhanced the model's accuracy.
Implications for Practitioners
The study's findings can aid practitioners in selecting the most relevant and impactful predictors for developing ML-based dementia risk prediction models. The authors note:
Knowing the predictive value of different predictor domains can aid practitioners in selecting the most appropriate predictors and informing them about the trade-offs involved in developing ML-based DRPMs tailored to their specific settings.![]()
Practical Challenges
While neuroimaging biomarkers offer high predictive value, they also pose challenges in terms of cost and accessibility. Brain MRI scans are expensive and may not be feasible for routine clinical care in the general population. Thus, practitioners must weigh the benefits against the practicalities of incorporating such advanced measures into their models.
Future Directions
Future research should explore other minimally invasive predictors, such as behavioral factors and blood-based biomarkers, to assess their unique contributions to predicting dementia risk. Additionally, examining these predictor domains across different age groups and populations can provide a more comprehensive understanding of their utility.
Conclusion
In summary, the study highlights the importance of a multidomain approach to predicting dementia. By combining various predictor domains, practitioners can develop more accurate and effective risk prediction models. Although advanced measures like neuroimaging biomarkers offer high predictive value, their practical challenges must be considered. The findings underscore the need for continued research and development in this field to better understand and predict dementia risk.
By leveraging the power of machine learning and multidomain data, we can take significant strides toward early detection and intervention, ultimately improving the lives of those at risk for dementia.