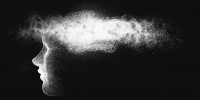
Machine Learning Uncovers New Alzheimer’s Biomarkers
A fresh study shows how machine learning is taking us in new directions in the identification of Alzheimer's biomarkers from cerebrospinal fluid. Using high-dimensional proteomics and a clever approach to handling small amounts of data, this research opens a new chapter in neurodegenerative diagnostics.
Alright, let's take a closer look at their findings!
Rethinking Alzheimer’s Diagnostics: What Machine Learning Found in the Proteome
In their recent publication in the journal Fluids and Barriers of the CNS, researchers from Chalmers University of Technology and University of Gothenburg explore the untapped potential of machine learning applied to high-dimensional TMT proteomics datasets.
And thus begins the search for new biomarkers in patients with idiopathic normal pressure hydrocephalus (iNPH)—a group that is unfortunately still often overlooked, but is of great importance for Alzheimer's research.
We propose eight protein and nine peptide biomarkers to differentiate iNPH patients across the pathological AD spectrum.![]()
This work doesn't just skim the surface. It tackles batch effects, missing data, and small cohorts with elegance.
And most importantly, it ultimately highlights potential biomarkers that could redefine the way we recognize Alzheimer's pathology.
At a Glance
Study Title: Applying machine learning to high-dimensional proteomics datasets for the identification of Alzheimer’s disease biomarkers
Published in: Fluids and Barriers of the CNS, 2025
Cohort: 106 iNPH patients, 186 cerebrospinal fluid (CSF) samples (lumbar & ventricular)
Key Tools: TMT proteomics, Random Forests, XGBoost, Logistic Regression, SMOTE, RFE
Top Finding: AUC of 0.84 achieved in classifying Alzheimer’s pathology from ventricular CSF
Proposed Biomarkers: GOT1, FABP3, MSTN, CAMK2G among others
Core Insight: Different CSF sample types (lumbar vs. ventricular) may require different biomarker strategies
Behind the Data: A Novel Lens on Neurodegeneration
Why iNPH Matters
Idiopathic Normal Pressure Hydrocephalus often mimics Alzheimer’s in symptoms, but its overlap remains under-investigated. This study leverages that overlap, analyzing CSF samples taken both via lumbar puncture and during surgery (ventricular). That dual-sample design is rare—and it sets the stage for some powerful insights.
The Machine Learning Pipeline: Designed for Complexity
When you’re working with high-dimensional, small sample proteomics data, most models break. Not here.
The team engineered a robust pipeline that:
Corrected for batch effects using the ComBat method
Handled missing values through comparison of imputation vs. feature removal
Balanced class distribution with SMOTE
Conducted feature selection using a consensus ensemble method from four different ML models
They didn't just stop at model accuracy—they checked feature stability across folds, ensuring that their biomarker suggestions weren’t flukes.
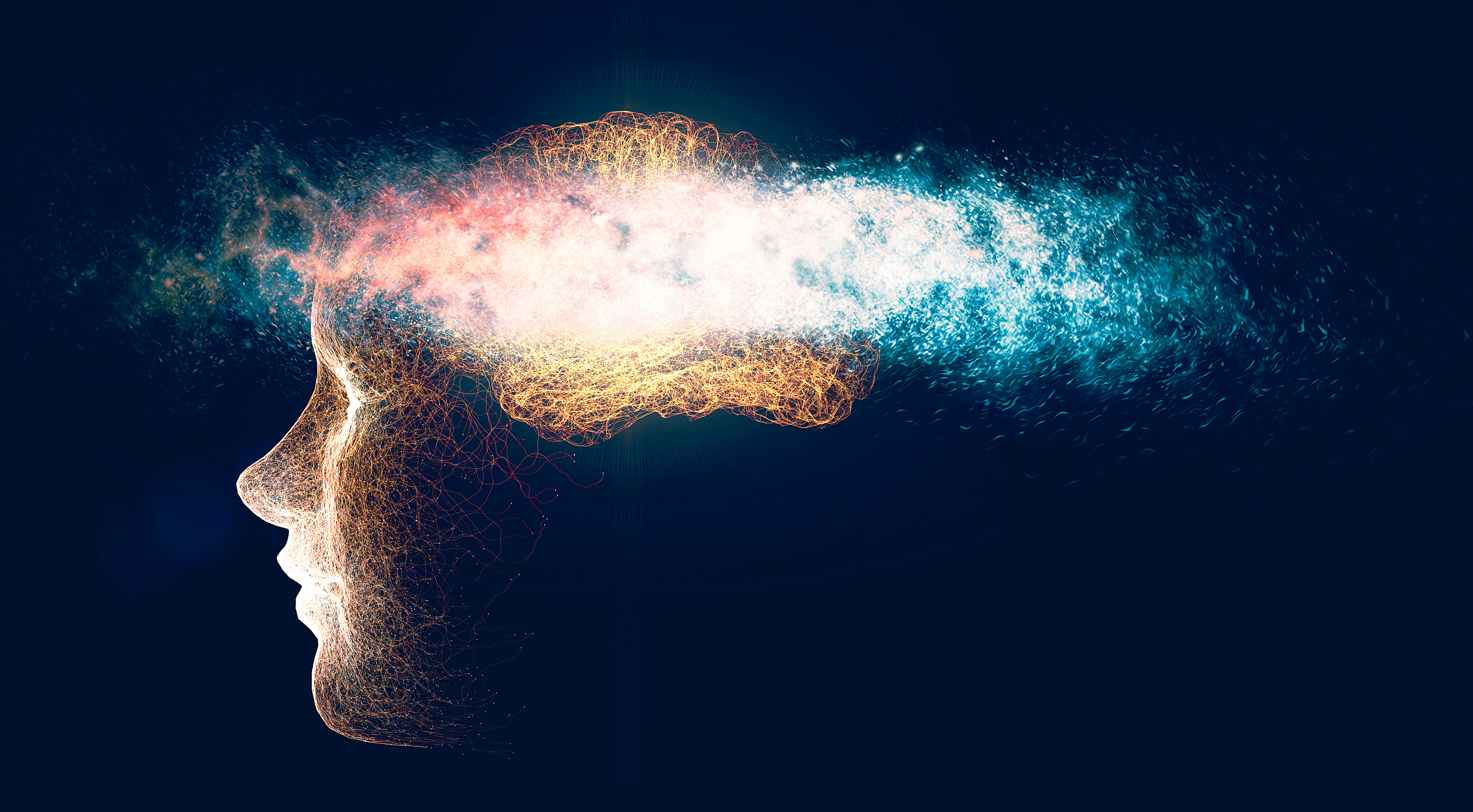
The Biomarkers That Emerged
From this meticulous setup, several proteins stepped into the spotlight:
FABP3: Known and reaffirmed—linked to amyloid pathology.
GOT1: A new contender, significantly elevated in patients with Alzheimer’s pathology in ventricular CSF.
MSTN and CAMK2G: Consistently selected across folds—possibly synergistic indicators.
Their takeaway? Some biomarkers behave differently depending on where in the CSF system the sample comes from. That’s a powerful call to rethink how and where we look.
A Closer Look at the Numbers
Random Forest (ventricular protein data):
AUC: 0.84 ± 0.03
F1-score: 0.58 ± 0.03
MCC: 0.46 ± 0.04
Notably, the team found that removing features with missing values performed better than imputing them. That’s a bold move in a field often quick to fill gaps with assumptions.
What This Means for the Field
Yes, there are many ML + proteomics papers. But here we almost have a blueprint on how to deal with the real complexity of omics data:
Embrace the small sample size—but be smart about it.
Don’t assume more data is better; sometimes fewer, cleaner features win.
Question the "universal biomarker" narrative. Context matters—CSF source matters.
And perhaps most importantly: innovative models can uncover what conventional methods overlook.
Final Thoughts
We would like to explain to you why we personally liked this study so much: When we discovered it and went through it, we simply felt inspired and a little more hopeful.
Because it reminded us once again of what we have known for a long time and what we at aimed analytics also work with: Data already provides answers if we just develop the right tools for listening.
And that is exactly what we do.