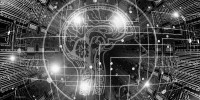
AI for Multi-Omics: Insights into Nano–Bio Interactions
Artificial intelligence is reshaping the study of nano–bio interactions by enabling complex data analysis, improving nanoparticle design, and personalizing treatments. With tools like machine learning, computer vision, and graph neural networks, AI unlocks insights that were previously unattainable.
Revolutionizing Nano–Bio and Multi-Omics Research with AI
A new perspective article published in Nature highlights how AI's ability to process large, complex datasets is critical for studying nano–bio interactions and multi-omics data. By integrating multi-omics datasets, analyzing imaging information, and predicting nanoparticle properties, AI simplifies processes that were once time-consuming and error-prone.
At a Glance
Artificial intelligence (AI) simplifies the analysis of complex, multi-omics datasets in nano–bio interactions.
Computer vision automates nanoparticle imaging analysis, enabling faster, precise insights.
Graph neural networks (GNNs) and deep learning optimize nanoparticle design and interactions.
Generative AI models predict nanoparticle properties and personalize therapies.
AI accelerates clinical decision-making by processing data faster than conventional methods.
The Role of Artificial Intelligence in Nano–Bio Interaction Studies
Artificial intelligence (AI) may greatly aid in the analysis of complex and big data sets, typical for multi-omics analyses, and may prove especially powerful if additional variables, such as nanoparticles, are added to the picture.![]()
Artificial intelligence (AI) is revolutionizing the analysis of large and complex datasets typical of multi-omics research. By incorporating additional variables such as nanoparticles, AI enhances our ability to investigate nano–bio interactions across diverse data types, offering unprecedented insights into biological systems.
Computer Vision for Image Analysis
The imaging of nanoparticles within labeled cells produces intricate datasets encompassing not only nanoparticles and cells but also components like blood vessels and macrophages. Manual quantification of such data is labor-intensive and prone to challenges in interpretation. AI, particularly computer vision combined with machine learning, can process and interpret visual data, identify patterns, and derive actionable insights.
For instance, computer vision techniques have been applied to assess nanoparticle delivery and biodistribution in mice, leveraging data from over 30 tumor models to evaluate how vascular permeability affects delivery efficiency. Similarly, machine learning has been used to quantify GFP signals in cells following GFP mRNA delivery via lipid nanoparticles, training models to predict GFP expression based on cell morphology and related parameters.
Big Data and Multi-Omics Integration
Multi-omics studies of nano–bio interactions involve integrating sequencing or mass spectrometry data with information on nanoparticle uptake and biodistribution. Deep learning models trained on these datasets can uncover drug action targets, identify cell types at the single-cell level, and reconstruct interaction networks.
Applying multi-omics data for the study of nano–bio interactions requires sequencing or mass spectrometry data of the cell to be organized and correlated in relation to nanoparticle uptake or distribution. Deep learning models, trained on multi-omics data, can be used to identify drug action targets or to distinguish cell types at the level of the individual cell.![]()
For example, DeepLinc reconstructs cell networks from spatial transcriptomics data, correlating molecular features with physiological or pathological states. Similarly, graph neural networks (GNNs) process complex biological networks such as protein–protein interactions, drug discovery pathways, and nanoscale interactions, aiding in protein-ligand affinity prediction and nanoparticle interaction modeling.
Emerging frameworks like scMoGNN combine single-cell sequencing data with biological knowledge, capturing higher-order structural relationships within cells, their environments, and nanoparticle characteristics. These advancements pave the way for designing nanoparticles tailored to specific cellular responses by integrating molecular data with protein and lipid libraries.
Predicting and Optimizing Nanoparticle Properties
Generative AI models, including reinforcement learning and generative adversarial networks (GANs), offer innovative approaches for nanoparticle design and optimization. These methods can be combined with clinical metadata (e.g., age, sex, and disease stage) to personalize nanoparticle-based therapies.
Reinforcement learning has been employed to predict nanoparticle cytotoxicity by analyzing extensive datasets of metal and metal-oxide nanoparticles, while GANs generate synthetic training data and simulate biological processes to enhance model performance. Supervised learning approaches have also been used to predict tumor nanoparticle accumulation, bridging the gap between biological factors and therapeutic delivery.
Accelerating Clinical Translation
AI's ability to rapidly process multi-omics data significantly reduces the time required for clinical decision-making. High-performance computing systems, including GPU clusters, accelerate gene sequencing analysis and multi-omics integration, enabling faster transitions from data collection to actionable insights.
For example, AI models streamline genome analysis, minimizing delays in clinical workflows and expediting the design of nanoparticle-based therapies tailored to patient-specific needs.
Designing Robust Multi-Omics Studies
Effective multi-omics studies must ensure reproducibility and robustness. Reliable labeling strategies, such as barcoding nanoparticles with DNA, RNA, peptides, or isotopes, allow for accurate detection across sequencing and mass spectrometry assays. Advanced imaging tools, including immune-labeled whole-body imaging and 3D tissue imaging, can visualize nanoparticle biodistribution, albeit with limitations in spatial and temporal resolution.
Accounting for variability in sex, age, genetic heterogeneity, and epigenetic factors is critical for consistent results in nano–bio interaction studies. Multi-omics technologies offer a path to understanding these factors at the single-cell level, revealing how spatial heterogeneity impacts nanoparticle interactions and cellular responses.
Outlook: Bridging Nano–Bio Mechanisms and Personalized Medicine
AI-powered multi-omics analyses hold immense potential for elucidating nano–bio interactions, improving nanoparticle design, and enabling personalized medicine. By combining transcriptomics, proteomics, and metabolomics, researchers can correlate nanoparticle formulations with cellular responses, shedding light on regulatory mechanisms and enhancing therapeutic efficacy.
Although challenges in nanoparticle imaging and biodistribution remain, advances in barcoding, sequencing, and super-resolution microscopy are providing new avenues for investigation. Integrating these tools with AI-driven analyses will likely uncover the mechanisms underlying nano–bio interactions, driving innovation in nanomedicine and personalized drug delivery platforms.