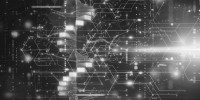
Overcoming Common Challenges in Omics Data Analysis
How can deep learning unravel the complexities of vast omics data to drive medical innovation? Dive into the strategies that are turning data challenges into eye-opening and opportunity-generating insights in the biological sciences.
Navigating the Challenges of Omics Data: Harnessing Deep Learning for Breakthroughs in Biological Research
At a Glance
Data Complexity and Volume: Omics technologies generate vast and complex datasets, necessitating robust computational infrastructure and data management strategies.
Multi-Omics Integration: Integrating diverse omics data types is challenging but essential for comprehensive biological insights. Standardization and advanced integration tools are key solutions.
Data Quality and Preprocessing: Ensuring data quality through rigorous quality control, normalization, and imputation methods is crucial for accurate analysis.
Statistical Analysis and Interpretation: Sophisticated statistical techniques are required to identify true biological signals amidst data noise.
Data Visualization: Effective visualization tools and pathway analysis are necessary for interpreting and communicating complex omics data.
Ethical and Privacy Concerns: Ensuring data privacy through anonymization and compliance with ethical guidelines is essential in omics research.
Role of Deep Learning: Deep learning offers promising solutions for omics data analysis but faces challenges in model interpretability and computational costs.
Introduction
Omics technologies have radically transformed biological research, offering comprehensive insights into genomics, transcriptomics, proteomics, metabolomics, and related fields. Despite their potential, the analysis of omics data presents numerous challenges. This article explores these challenges and suggests strategies to overcome them, with a focus on the role of deep learning in the omics field.
The widespread availability of complex and unstructured omics data presents both enormous potential and significant challenges for the healthcare research field. As Mohammed et al. (2023), for example, note in their review, understanding and leveraging this data effectively is crucial for advancements in precision medicine and disease treatment.
The Complexity and Volume of Data
Omics data are characterized by their vast volume and complexity. High-throughput sequencing and mass spectrometry technologies generate datasets with millions of data points, posing significant challenges in terms of storage, management, and analysis.
But this is where AI and machine learning algorithms can help with regard to the gigantic amount of data:
One of the most exciting emerging trends is the integration of AI and ML with multi-omics data analysis. AI and ML algorithms can handle the complexity and volume of multi-omics data, identifying patterns and correlations that would be challenging for human analysis alone.![]()
Solutions:
High-Performance Computing (HPC): Leveraging HPC systems can expedite data processing and analysis. Cloud-based platforms like AWS and Google Cloud offer scalable solutions that are well-suited to handling large omics datasets.
Data Compression and Reduction Techniques: Employing data compression algorithms and dimensionality reduction techniques, such as Principal Component Analysis (PCA), can simplify data handling and analysis.
AI and Machine Learning: These technologies enable the discovery of novel insights by analyzing large datasets with precision and speed that surpass traditional methods.
Integration of Multi-Omics Data
Integrating data from various omics layers is crucial for a comprehensive understanding of biological systems. However, differences in data types, formats, and scales pose significant integration challenges.
Solutions:
Standardization of Data Formats: Initiatives like the FAIR (Findable, Accessible, Interoperable, Reusable) data principles promote data standardization and interoperability, facilitating easier data integration.
Advanced Data Integration Tools: Tools such as Multi-Omics Factor Analysis (MOFA) and iCluster can identify patterns across different omics layers, providing unified insights.
Data Quality and Preprocessing
The quality of omics data can be compromised by experimental errors, batch effects, and missing values, potentially leading to inaccurate results and interpretations.
Solutions:
Rigorous Quality Control: Implementing stringent quality control measures, including the use of control samples and replicates, can help identify and mitigate potential errors.
Data Normalization and Batch Effect Correction: Techniques such as quantile normalization and ComBat can correct batch effects, ensuring data comparability across experiments.
Imputation of Missing Values: Missing data can be addressed using imputation methods like k-nearest neighbors (KNN) and multiple imputation, which estimate missing values based on observed data patterns.
Statistical Analysis and Interpretation
The statistical analysis of omics data is challenging due to the high dimensionality and inherent noise in the datasets. Identifying true biological signals amidst the noise requires sophisticated statistical techniques.
Solutions:
Robust Statistical Methods: Employing advanced statistical methods such as Bayesian inference, machine learning algorithms, and differential expression analysis can help uncover meaningful patterns in the data.
Multiple Testing Correction: Applying multiple testing correction methods, such as the Benjamini-Hochberg procedure, can control the false discovery rate, reducing false positives.
Biological Validation: Statistical findings should be corroborated with experimental validation to ensure their biological relevance.
Data Visualization and Interpretation
Visualizing omics data is essential for interpreting complex results and communicating findings effectively. However, the multidimensional nature of the data complicates visualization efforts.
Solutions:
Advanced Visualization Tools: Utilizing tools such as R's ggplot2, Python's Matplotlib, and specialized software like Cytoscape can aid in creating informative and interactive visualizations.
Network and Pathway Analysis: Visualizing data within the context of biological networks and pathways can provide insights into the functional implications of the findings.
Ethical and Privacy Concerns
The increasing availability of omics data raises ethical and privacy concerns, particularly when dealing with human data. Ensuring the confidentiality and proper use of this data is crucial.
Solutions:
Data Anonymization: Implementing data anonymization techniques can protect individual privacy while allowing for meaningful analysis.
Ethical Guidelines and Compliance: Adhering to established ethical guidelines and regulatory frameworks, such as the GDPR in Europe and HIPAA in the United States, is essential for responsible data handling.
The Role of Deep Learning in Omics
Recent advances in deep learning (DL) have shown promise in overcoming some challenges in omics data analysis. These deep learning models have been increasingly applied to omics data, offering new ways to integrate and interpret complex datasets. However, several challenges, such as model interpretability, data scarcity, and computational costs, still need to be addressed.
The healthcare research field has enormous potential and problems because of the widespread availability of complex and unstructured omics data.![]()
Solutions:
Model Interpretability: Developing interpretable DL models is crucial for their acceptance in clinical settings. Approaches like attention mechanisms and explainable AI can help elucidate the decision-making process of DL models.
Handling Data Scarcity: Transfer learning and data augmentation techniques can mitigate the issue of data scarcity, enabling DL models to learn effectively from limited datasets.
Reducing Computational Costs: Optimizing DL architectures and utilizing hardware accelerators, such as GPUs and TPUs, can reduce computational costs and improve model efficiency.
Conclusion
Despite the challenges associated with omics data analysis, advances in computational tools, statistical methods, and data integration strategies continue to enhance our ability to extract meaningful insights from complex biological data.
By addressing these challenges and harnessing the power of deep learning, researchers can unlock the full potential of omics technologies, paving the way for new discoveries and advancements in biomedical research.
Understanding and overcoming these challenges is crucial for success in the ever-evolving landscape of omics research. As the field continues to grow, interdisciplinary collaboration and innovative solutions will be key to overcoming these obstacles and advancing our understanding of life at the molecular level.