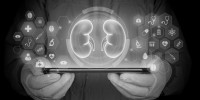
Unlocking Precision in Proteomic Analysis
Analyzing large-scale proteomics data presents a complex challenge for analytical chemists, especially in the context of varied data sources and significant variability. Bioinformatics tools are crucial, yet often, their application is hindered due to underdeveloped training in these areas. To address this, researchers are exploring normalization methods to effectively manage the inherent variability and enhance data comparability.
Unveiling Shotgun-based Proteomics Workflows
Shotgun-based proteomics workflows are spotlighted for their multifaceted variability sources: sampling, handling, storage, and mass spectrometry measurements. These discrepancies necessitate reliable normalization methods to extract meaningful insights from the data.
Normalization for Enhanced Comparability
Normalization, a technique adjusting data from different sources, eliminates undesired variability and boosts comparability. The choice of normalization method holds the key to downstream analysis quality. Three prominent normalization methods take center stage:
Z-Score Normalization: This method reshapes data by subtracting each protein value's median across all samples and dividing by the protein's standard deviation. It transforms data into a normal distribution, beneficial for rigorous statistical analyses.
Median Divide Normalization: In this approach, all data points are divided by the median value, curtailing bias arising from equipment variations. While fitting for extensive datasets across time, it might not suit extreme values or data clusters near zero.
Quantile Normalization: Here, each data point is replaced with the mean of the corresponding quantile, homogenizing data distribution across samples. This method proves valuable for comparing datasets with diverse distributions.
Normalization is a method used to adjust the data obtained from different samples, platforms, or batches to remove unwanted variability and enhance comparability between the datasets. It involves applying a mathematical correction to the data to reduce the effects of systematic technical variation and other sources of bias.![]()
A Case Study: Evaluating Normalization Techniques in Renal Carcinoma Research
In a recent study, these normalization methods were put to the test using proteomic data from various renal carcinomas and normal adjacent tissue samples. The primary goal was to assess how different normalization techniques impact the identification of significant proteins and downstream analyses. The findings of this study were then compared to previously validated tissue microarrays.
Methodology in a Nutshell
The research encompassed patients and sample collection, mass spectrometry data generation, protein identification and quantification, and meticulous bioinformatics data processing. The latter involved eliminating irrelevant protein groups, annotating sample conditions, transforming data, and imputing missing values. The selected normalization methods were applied, and their influence on downstream analyses, including protein-protein interactions and biochemical pathways, was meticulously examined.
Insights Derived
The results uncovered valuable insights:
For two-group comparisons, Z-score normalization or quantile normalization are recommended, as they excel in identifying differentially expressed proteins.
When comparing multiple groups (3, 4, or 5 datasets), the choice of normalization method has minor impact, allowing flexibility in method selection.
The study underscored the significance of the Total Protein Approach (TPA) in biomarker discovery. Some biomarkers, previously identified using traditional methods, were only highlighted due to the robustness of TPA.
Enhancing Precision Medicine
By delving into different subtypes of renal tumors and control groups, this study contributes to the realm of precision medicine-focused research. It reaffirms the necessity of meticulous normalization techniques for accurate and reproducible proteomic analyses.
Conclusion: The article delves into the critical role that normalization methods play in navigating the complexities of proteomics data. By shedding light on their impact on analyses and identification of biomarkers, it underscores the importance of careful method selection. The study not only advances proteomics research but also amplifies the significance of alternative methodologies like TPA. In an era of precision medicine, unlocking the power of normalization methods propels us closer to more targeted and effective medical solutions.