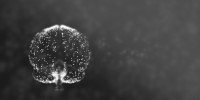
Redefining Stroke Diagnosis: Integrative Multi-Omics
Today, we take a look at the future of stroke treatment with recent research that merges high-tech omics data and cutting-edge Graph Neural Networks. Discover how this innovative approach is unearthing new biomarkers and revolutionizing stroke diagnosis, paving the way for personalized medicine.
The Stroke Conundrum: Current Challenges and New Horizons
At a Glance
A few exciting and important points from the research article first:
Revolutionary Insight: Leveraging multi-omics data integration to classify stroke types and discover novel biomarkers.
Key Technology: Use of Graph Neural Networks (GNNs) to handle complex biological data.
Breakthrough Discovery: Identification and validation of a potential biomarker; namely circRNA hsa_circ_0005568, validated by Real Time Quantitative PCR (RT-qPCR), an amplification method for nucleic acids.
Superior Performance: BioMGNN model outperformed traditional methods in classifying stroke etiology and identifying biomarkers.
Future Potential: This approach could significantly enhance personalized treatment strategies for stroke patients.
Understanding the Stroke Challenge
Stroke is a leading cause of disability and death globally. Despite advancements, pinpointing the exact cause of many strokes remains challenging. Traditional diagnostic methods, relying heavily on clinical evaluations and imaging, still leave about 20-25% of stroke cases with undetermined causes. That’s where high-throughput omics and data integration come into play, offering a more detailed molecular perspective that could revolutionize stroke treatment and diagnosis.
The Importance of Biomarkers
Biomarkers are essential for accurately diagnosing and classifying strokes, which can guide better treatment strategies. They provide objective data that can predict clinical outcomes and monitor therapeutic responses. Multi-omics approaches, which combine various biological data types, promise to offer a holistic view of the molecular underpinnings of stroke, leading to the discovery of reliable biomarkers.
Methods for Multi-Omics Data Integration
Several strategies have been developed to integrate multi-omics data:
Correlation Analysis: Looks at how different omics datasets co-vary, revealing potential regulatory mechanisms.
Dimensionality Reduction: Techniques like PCA and ICA simplify complex data, making it easier to integrate.
Integrative Clustering: Identifies subgroups of samples by considering similarities across various omics layers.
Autoencoders: Neural networks that learn compressed representations of the data.
Graph Neural Networks (GNNs): Utilize graph structures to analyze multi-omics data, capturing intricate interactions.
A Graph-Based Approach
In this study, researchers introduce a novel method that leverages Graph Neural Networks (GNNs) to integrate multi-omics data. By constructing a knowledge graph that depicts relationships between various molecular components, the model can better capture complex interactions and improve the accuracy of stroke etiology classification.
Study Design
Participants
The study focused on 30 acute ischemic stroke patients from the Hospital of Navarra, categorized into different etiological subgroups using the TOAST criteria. Blood samples were collected within 24 hours of admission, and a comprehensive set of diagnostic tests was performed.
Data Collection
mRNA Expression: Assessed using Agilent microarrays.
MicroRNA (miRNA) Expression: Analyzed with the miRCURY LNA™ microRNA Array.
Circular RNA (circRNA) Expression: Detected using Arraystar Human circRNA Array.
DNA Methylation: Profiled with the Infinium HumanMethylationEPIC BeadChip array.
Integrating the Data
Building the Knowledge Graph
Researchers created a biomedical knowledge graph to map the interactions between different omics datasets and patient data. This graph formed the backbone of their Graph Neural Network (GNN) analysis.
Constructing the GNN Model
The BioMGNN model integrates multiple omics layers and uses graph convolutional operations to propagate information through the network. The model was trained using PyTorch Geometric and optimized with an Adam optimizer, running on powerful NVIDIA GPUs.
Results
Classifying Patients
The BioMGNN model showed superior performance in classifying stroke etiology compared to traditional single-omics approaches. It effectively clustered similar patients and improved prediction accuracy.
Discovering Biomarkers
The GNN identified significant node interactions, highlighting potential biomarkers. One notable finding was the differential expression of circRNA hsacirc0005568, which was validated using RT-qPCR.
Comparing with Other Methods
The BioMGNN outperformed other multi-omics tools like MOFA and MOGONET in biomarker discovery and patient classification, showcasing its potential in precision medicine.
Discussion
While the initial results are promising, the study acknowledges the need for further validation with larger, more diverse cohorts. Future research will focus on refining the GNN architecture, expanding data integration, and testing the model in broader patient populations.
Conclusion
The integrative multi-omics approach using Graph Neural Networks offers a powerful tool for understanding stroke etiology and discovering reliable biomarkers. With further validation, this method could significantly enhance personalized medicine and treatment strategies for stroke patients.
Looking Ahead
Optimizing Graph Networks: Explore advanced architectures and training techniques.
Expanded Data Integration: Incorporate additional omics data and external databases.
Clinical Validation: Test the model in larger, more diverse patient cohorts.
User-Friendly Tools: Develop accessible libraries for researchers to apply this method in their studies.
By harnessing the latest advancements in artificial intelligence and multi-omics integration, researchers are paving the way for more precise, individualized treatment options for stroke patients. This study marks a significant step towards that goal.