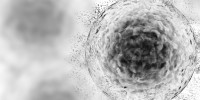
Revolutionizing Stem Cell Research with AI and Imaging
Unlock the secrets of stem cell behavior with groundbreaking research from USC. Utilizing machine learning and innovative imaging, this study offers unprecedented, non-invasive insights that could revolutionize regenerative medicine and stem cell therapies.
Revolutionizing Stem Cell Research with Machine Learning and Imaging
New research from the University of Southern California’s Alfred E. Mann Department of Biomedical Engineering has unveiled a groundbreaking non-invasive system to study stem cell behavior. Led by Associate Professor Keyue Shen, the team has harnessed the power of machine learning and imaging to provide unprecedented insights into how stem cells proliferate and regenerate into specialized cells.
This innovative approach has the potential to significantly advance drug discovery, stem cell treatments, and regenerative medicine.
At a Glance
Research Focus: Understanding stem cell proliferation and differentiation
Lead Researcher: Associate Professor Keyue Shen
Institution: University of Southern California
Published In: Science Advances
Key Technology: Fluorescence lifetime imaging microscopy (FLIM)
Applications: Stem cell treatments, drug discovery, regenerative medicine
The Holy Grail of Medicine
Stem cells serve as the body’s emergency toolkit, capable of transforming into various specialized cells, such as immune cells and brain cells. They possess the unique ability to divide and regenerate indefinitely, playing a crucial role in repairing and replenishing tissues and organs.
The ability to culture stem cells in the lab and direct them to become any cell type needed is considered the Holy Grail of medicine. Achieving this could enable clinicians to maintain an endless supply of new cells for repairing damaged tissues and organs.
A Non-Invasive Approach
Shen’s research primarily focuses on hematopoietic stem cells, which reside in bone marrow and give rise to all blood cells, including red blood cells and immune cells. Using fluorescence lifetime imaging microscopy (FLIM), the team tracks the metabolism of these stem cells in real-time. This method leverages the natural autofluorescence of cellular molecules like NADH to measure metabolic activity without destroying the cells.
It's very exciting because we are not killing the cells. We are merely just taking images of the cell and then extracting those features. That can give us so much information about them![]()
Machine Learning Insights
By employing a machine learning approach, the researchers developed a library of 205 metabolic optical biomarker features, 56 of which are associated with hematopoietic stem cell differentiation. This allows them to create a clustering map to distinguish between stem cells and non-stem cells, as well as track their behavior and differentiation over time.
The system assigns a score to predict whether a daughter cell will retain stem cell properties or differentiate into a specific cell type.
Real-Time Understanding of Stem Cell Metabolism
The team's real-time imaging approach provides foundational knowledge that could revolutionize cell therapy and regenerative medicine. For instance, in bone marrow transplants, achieving symmetric division of stem cells could generate a large stock of cells for multiple patients. Currently, blood stem cells cannot be expanded outside the body in clinical settings, but this research brings us closer to solving this challenge.
Broader Applications
Shen’s pioneering work opens new avenues for various medical applications, including stem cell therapy and regenerative medicine. It allows scientists to monitor the state of stem cells in real-time and track their transitions over time, which was previously not possible. This breakthrough could lead to significant advancements in creating specialized cells for treating a wide range of diseases.
Conclusion
The integration of machine learning and imaging in stem cell research is a significant leap forward. Shen’s innovative approach provides a detailed, non-invasive method to study stem cell behavior, offering new possibilities for medical treatments and stem cell therapies.
This research not only enhances our understanding of stem cell metabolism but also paves the way for future advancements in regenerative medicine.