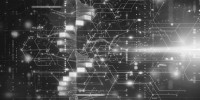
Simplifying Bioinformatics for Academic Researchers
Unlock the full potential of your biomedical data without the complexity. Today’s bioinformatics platforms bring the power of AI, machine learning, and multi-omics integration to academic researchers, helping streamline analysis and deepen insights. With advanced tools to handle genomics, transcriptomics, epigenomics, proteomics, and more, researchers can make impactful discoveries faster and more easily.
Enhancing Bioinformatics with AI and Omics Technologies
At a Glance
Seamless Multi-Omics Integration: Combine genomics, transcriptomics, epigenomics, and proteomics for richer insights.
AI and Machine Learning-Powered Analysis: Accelerate research with predictive and analytical tools that learn from data patterns.
User-Friendly, Accessible Platforms: Reduce the technical barriers of bioinformatics with intuitive interfaces.
Enhanced Data Visualization and Collaboration: Visualize complex data and collaborate in real time for more efficient research.
The Evolving Need for Simplified Bioinformatics in Academia
Bioinformatics is foundational for understanding the biological mechanisms behind human health and disease, yet the technical challenges of handling complex data often slow down research in academic settings. Advanced tools and AI-powered platforms are now helping to lower these barriers, making bioinformatics accessible for a broader range of researchers. With solutions that integrate AI, machine learning, and multi-omics data, academic researchers can focus on discovery rather than struggling with technical configurations.
AI has become an integral part of bioinformatics research, having deeply embedded in many aspects of the field. Among various AI algorithms, Deep learning (DL) has gained significant popularity in bioinformatics and is applied more frequently in various tasks compared to other traditional methods.![]()
How AI is Revolutionizing Bioinformatics
Enhancing Data Analysis with AI and Machine Learning
Artificial intelligence and machine learning are game-changers for data analysis, offering automated pattern recognition and predictive modeling capabilities that were previously unattainable. By processing large datasets, such as those produced in high-throughput sequencing, machine learning algorithms help researchers identify correlations and anomalies, accelerating their ability to generate hypotheses and identify new biological insights.
Key Benefits of AI, Deep Learning and Machine Learning in Bioinformatics:
Automated Pattern Recognition: Quickly identify correlations and patterns within complex data.
Predictive Analytics: Model biological outcomes, invaluable in fields like pharmacogenomics and disease prediction.
Anomaly Detection: Flag unusual data points for further investigation, aiding in early disease detection.
With AI-powered models, academic researchers can conduct data analysis that’s faster, more accurate, and more insightful, even without a background in computational science. Deep learning specifically enables researchers to detect subtle trends across complex datasets by using artificial neural networks with multiple layers. This is particularly powerful in bioinformatics applications where data is high-dimensional, such as in imaging or sequencing data, where deep learning’s ability to process and recognize fine-grained patterns leads to more accurate results.
The Role of Transcriptomics in Biomedical Research
Transcriptomics—the study of RNA transcripts—provides a snapshot of gene expression in cells, tissues, or organisms at any given time, offering invaluable insights into cellular activity and the regulation of gene expression. Specialized transcriptomics platforms allow researchers to analyze RNA sequencing (RNA-seq) data, revealing which genes are active, to what extent, and how gene expression changes in response to different conditions.
For academic researchers, transcriptomics plays a critical role in exploring gene regulation, understanding disease mechanisms, and identifying biomarkers for drug development. By studying RNA profiles, researchers can, for example, differentiate disease subtypes, track disease progression, or identify gene expression patterns associated with drug responses. AI-driven transcriptomics solutions make it possible to analyze these vast datasets efficiently, clustering expression data, detecting changes across conditions, and identifying subtle expression patterns that might signal disease onset or progression.
Platforms that specialize in transcriptomic data solutions offer enhanced tools for RNA analysis, tailored workflows for RNA-seq data processing, and powerful visualization capabilities. These platforms make it easy for researchers to uncover gene expression dynamics, accelerating discoveries in fields such as cancer research, neurobiology, and immunology.Understanding Multi-Omics and Its Applications
Each omics layer offers unique information about biological systems, but combining them provides a more comprehensive picture. This is where multi-omics approaches come in, integrating data from genomics, transcriptomics, epigenomics, proteomics, and metabolomics to reveal deeper insights into how molecular processes interact within the body. AI plays a crucial role in multi-omics by integrating diverse datasets and enabling holistic analysis.
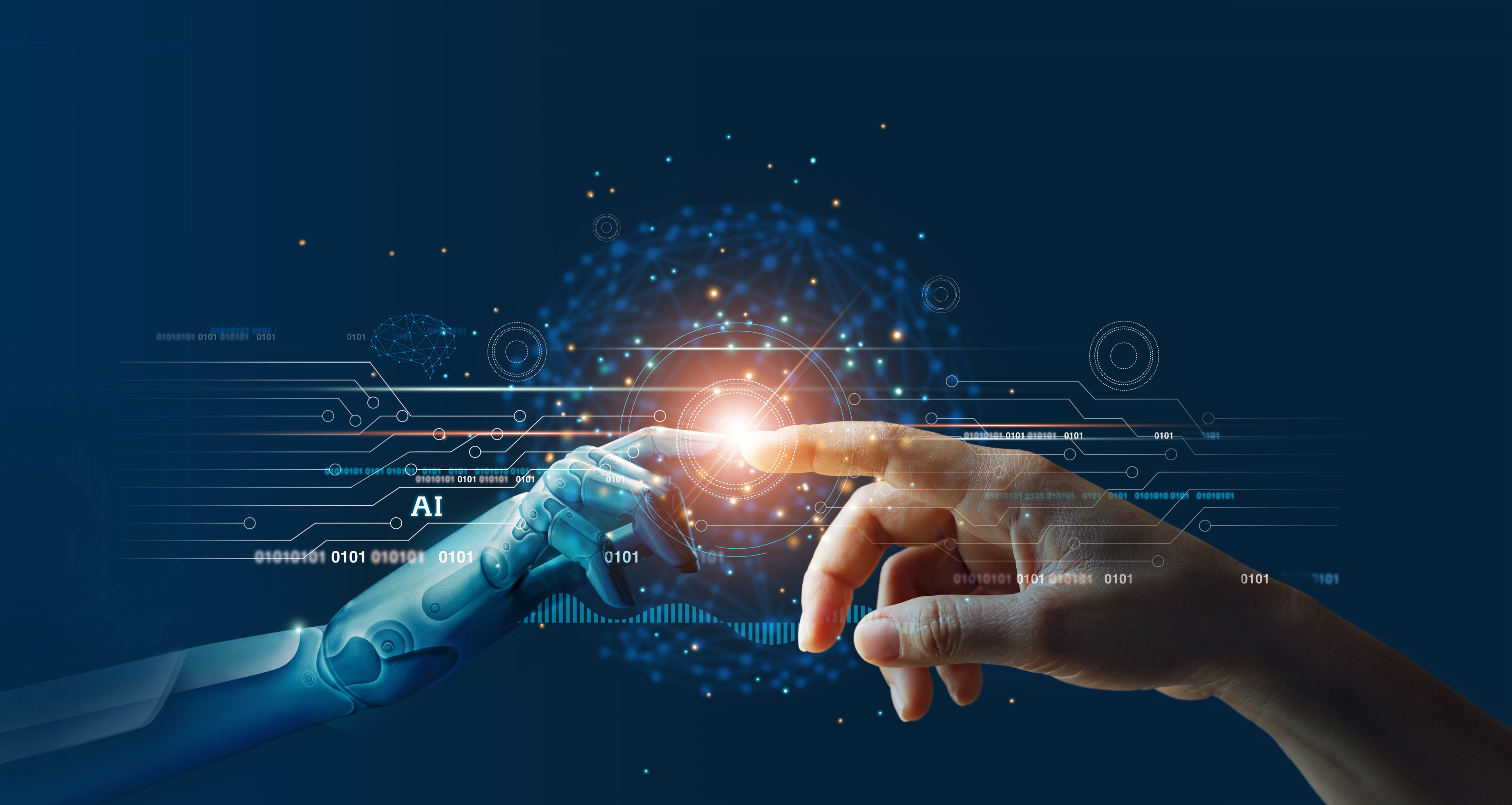
The Key Omics Layers and How AI Supports Them
1. Genomics
Genomics analyzes an organism’s complete DNA sequence, encompassing genes and regulatory regions. AI-driven analysis has transformed genomics, particularly in variant analysis and genome annotation, by speeding up identification of disease-associated mutations and structural variants.
2. Transcriptomics
Focusing on the transcriptome (the complete set of RNA molecules), transcriptomics reveals which genes are actively expressed. Machine learning aids researchers by clustering gene expression patterns, detecting subtle changes that can indicate disease states and highlight potential biomarkers.
3. Epigenomics
Epigenomics explores modifications to DNA that influence gene expression without altering the sequence itself. AI helps uncover these complex modifications, such as DNA methylation and histone modification, which play key roles in processes like cancer progression and aging.
4. Proteomics
Proteomics studies proteins, the main effectors of biological processes. AI assists in analyzing mass spectrometry data to identify protein structures and functions. In drug discovery, AI helps map protein-protein interactions, revealing how proteins work together and identifying potential therapeutic targets.
5. Metabolomics
Metabolomics focuses on the small molecules produced during cellular metabolism, providing a snapshot of cellular health. Machine learning algorithms make it easier to classify and analyze metabolomic data, which can reveal metabolic shifts associated with diseases.
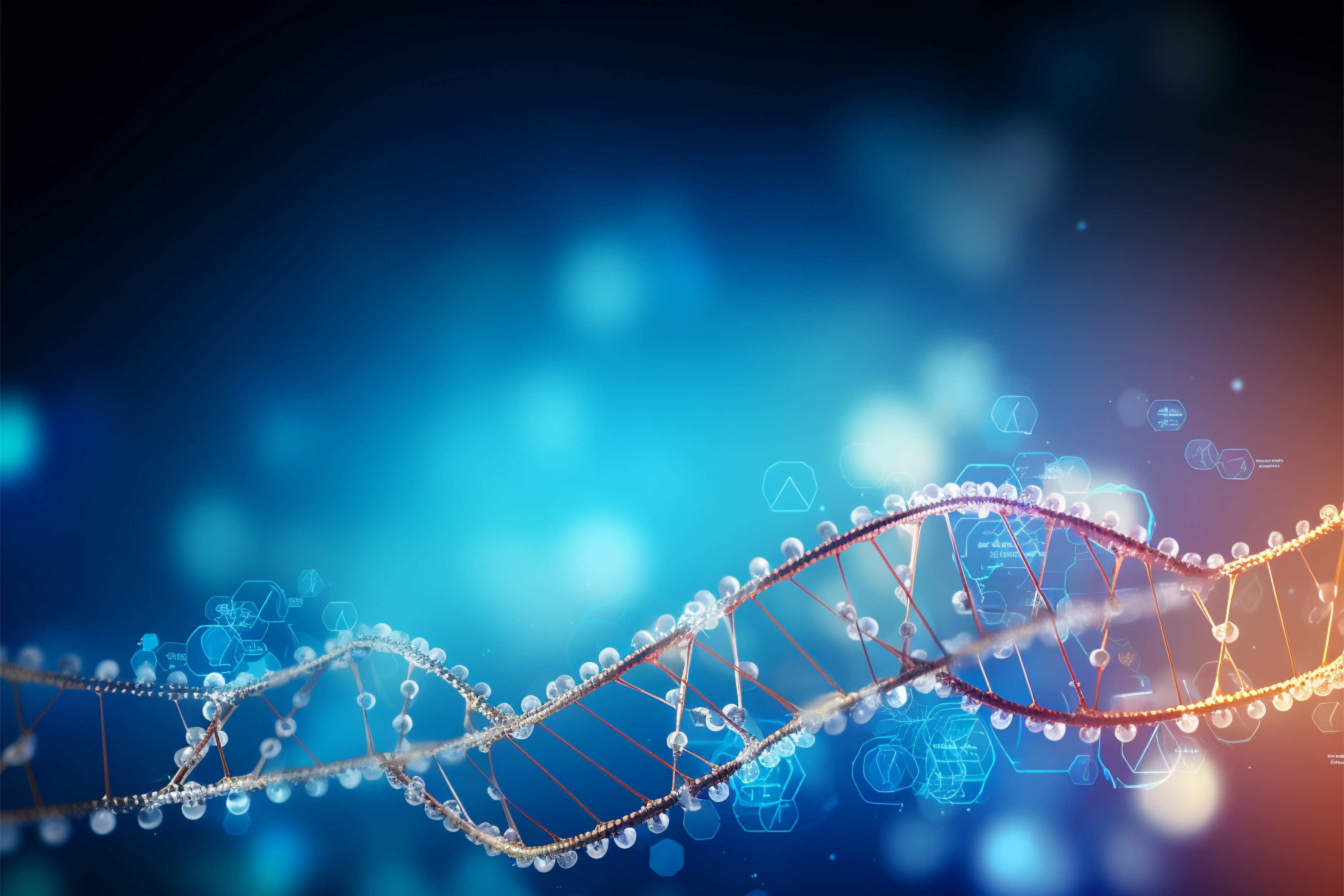
How Multi-Omics Enhances Biomedical Research
Multi-omics approaches enable researchers to see the complex interplay between genes, proteins, and metabolites, which provides richer context and insights into biological systems. For instance, cancer research can benefit from multi-omics by integrating genomics, transcriptomics, and proteomics data to reveal tumor mechanisms, offering opportunities for targeted therapies.
Examples of Multi-Omics Applications
Cancer Research and Precision Medicine
By combining multiple omics data layers, researchers can identify genetic and protein interactions that drive tumor growth, leading to more precise cancer subtypes and personalized therapies.Drug Discovery and Development
Multi-omics analysis helps identify how genetic and metabolic factors affect cellular responses to drugs. AI-driven multi-omics platforms expedite drug discovery by predicting which compounds are most likely to succeed based on their effects across omics layers.Chronic and Complex Disease Research
Multi-omics offers insights into how genetic and environmental factors interact in complex diseases like diabetes and cardiovascular disease, allowing researchers to identify risk factors and potential intervention points.
User-Friendly Tools for All Researchers
Not all academic researchers have a bioinformatics background, so modern platforms have evolved to provide intuitive, user-friendly interfaces that make data analysis accessible to all. Features like drag-and-drop functionality, interactive visualizations, and simplified workflows reduce technical complexity and allow researchers to focus on analysis.
Features of User-Friendly Bioinformatics Platforms:
Drag-and-Drop Tools: Enable easy data import and manipulation without coding.
Interactive Visualizations: Help explore datasets visually, which is especially useful for identifying trends.
Collaboration Tools: Facilitate team-based research, allowing real-time data sharing and workflow management.
By reducing the technical demands of bioinformatics, these tools help academic researchers across disciplines unlock the power of their data without needing extensive computational training.
Key Advantages of AI-Driven Multi-Omics for Academic Researchers
Holistic Insights: Multi-omics provides a multi-dimensional view of biological systems, allowing researchers to explore relationships between genes, proteins, and metabolites.
Accelerated Discoveries: Machine learning algorithms rapidly analyze complex datasets, offering faster results and helping researchers generate data-driven hypotheses.
Reduced Complexity: User-friendly platforms and AI support make bioinformatics accessible to non-specialists.
Enhanced Precision Medicine: AI-powered models can leverage omics data to tailor treatments based on individual molecular profiles, paving the way for personalized therapies.
Conclusion
Bioinformatics platforms that combine AI, deep learning, machine learning, and multi-omics capabilities are reshaping the field for academic researchers. By eliminating technical barriers and integrating powerful computational tools, these platforms help researchers focus on breakthroughs, not back-end complexity. Whether analyzing genomic sequences or exploring protein networks, researchers can now gain deep insights into biological processes, leading to faster, more precise discoveries and enabling the development of more personalized and effective treatments.