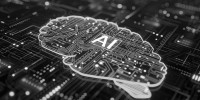
Spatial Omics: AI's Role in Biomedical Breakthroughs
Discover how the integration of Artificial Intelligence (AI) with cutting-edge spatial omics technologies is revolutionizing biomedical research. From single-cell precision to comprehensive 3D tissue mapping, AI is unlocking new dimensions of data analysis, paving the way for groundbreaking discoveries and personalized medicine.
Unlocking the Power of Spatial Omics with AI
A recent comment published in Nature Methods by Kyle Coleman, Amelia Schroeder, and Mingyao Li delves into the transformative potential of spatial omics technologies and how AI could unlock their full power. The comment discusses the remarkable advancements in spatial omics and the substantial challenges that remain, and how AI can facilitate the integration of complex datasets to foster further biomedical breakthroughs.
At a Glance
Spatial Omics Revolution: Offering unprecedented insights into tissue structure and function.
AI Integration: Facilitating the integration of complex datasets for groundbreaking discoveries.
Single-Cell Precision: Combining sequencing and imaging techniques for comprehensive spatial transcriptomics.
Multi-Omics Data: AI could generate spatial multi-omics data in silico, reducing experimental costs.
3D Tissue Mapping: AI enables the construction of detailed 3D spatial molecular maps.
Future of Medicine: Multi-modal large language models (MM-LLMs) hold promise for precision medicine and personalized treatments.
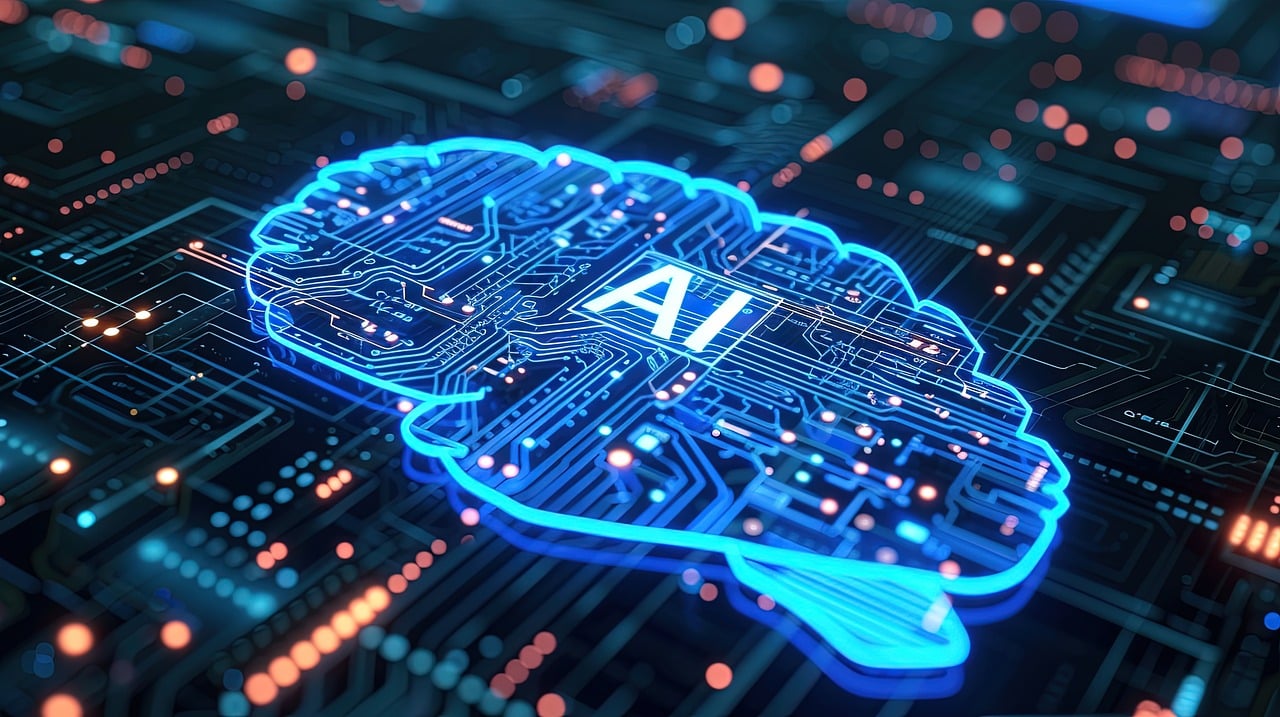
Increasing Spatial Resolution and Gene Coverage
Recent advancements such as Visium HD and Stero-seq1 have enabled sequencing-based spatial transcriptomics to measure gene expression across the entire transcriptome at subcellular resolution. Despite this precision, these methods face challenges in profiling single cells in situ due to a lack of cell boundary information. Conversely, imaging-based spatial transcriptomics technologies like MERFISH2, CosMx3, and Xenium offer single-cell level measurements but are limited to profiling only a few thousand genes per tissue section.
To address these limitations, AI-driven approaches are proposed to combine imaging-based and sequencing-based spatial transcriptomics with single-cell RNA sequencing (scRNA-seq). This integration could achieve comprehensive, single-cell-resolution spatial transcriptomics data, facilitating the identification of rare cell types and characterizing complex cell interactions.
Facilitating Spatial Multi-Omics Data Generation
Spatial multi-omics technologies have made it possible to profile multiple spatially resolved omics modalities from a single tissue section. However, the extraction of data for one modality can affect the molecular integrity required for another, reducing sensitivity in subsequent rounds of sequencing or imaging.
AI can be leveraged to generate spatial multi-omics data in silico, profiling a broader array of modalities than is feasible experimentally. By extracting multiple adjacent tissue sections and profiling them using different techniques while maintaining an anchor modality, like hematoxylin & eosin (H&E) staining, AI models can infer unobserved omics modalities based on established patterns. This approach could significantly reduce costs and complexities associated with generating comprehensive datasets.
3D Reconstruction of Tissue Architecture
Most spatial omics technologies provide molecular measurements at 2D spatial coordinates within a single tissue section, typically ~5 µm in depth. This limitation is critical when studying diseases like cancer, where a single 5-µm section offers a very limited view of cellular interactions and tumor structure.
AI methods are essential for constructing a complete 3D spatial molecular map of a tissue block. By generating spatial omics and H&E imaging data for selected tissue sections and using AI to infer spatial omics data for unmeasured sections, researchers can achieve a pseudo-3D super-resolution spatial multi-omics dataset. This would provide a more comprehensive understanding of tissue architecture and disease progression.
Enhancing Spatial Omics with Multi-Modal Large Language Models
Multi-modal large language models (MM-LLMs) have shown promise in tasks related to pathology imaging, scRNA-seq, and spatial transcriptomics. By leveraging large collections of spatial omics datasets, MM-LLMs can enhance resolution and coverage of spatial omics data and infer unmeasured omics modalities. These models can also be used for tasks such as spatial domain detection, spatially variable gene detection, and cell-cell communication analysis.
The potential of MM-LLMs extends to precision medicine, where spatial omics technologies offer insights into the cellular and molecular organization of disease-relevant structures. By correlating spatial molecular patterns and pathology imaging features with clinical outcomes, these models can help develop personalized treatment plans.
Conclusion
Despite significant strides in spatial omics technologies, persisting limitations highlight the essential role of AI in advancing this field. AI can facilitate the integration of spatial transcriptomics with scRNA-seq, construct high-resolution 3D spatial atlases of tissues, and train MM-LLMs for tasks in biomedical research and precision medicine. By harnessing the power of AI, researchers can unlock new dimensions of spatial omics, leading to groundbreaking discoveries and better health outcomes.
As the authors succinctly put it:
Artificial intelligence (AI) presents promising solutions that can potentially transform these challenges into opportunities to fully unlock the power of spatial omics for further breakthroughs.![]()