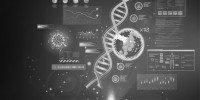
The Synergy of AI and Medicine
Dive into the transformative realm where artificial intelligence meets medicine. From personalized disease treatment to streamlined clinical trials, discover how AI, driven by data, is revolutionizing patient care and reshaping the landscape of modern medicine. Join us on a journey through the innovative applications, benefits, and future prospects of this powerful alliance.
Data-Driven Healthcare Revolution: Unveiling the Synergy of AI and Medicine
How does artificial intelligence contribute to the field of medicine? In the realm of healthcare, artificial intelligence leverages machine learning models to sift through vast amounts of medical data, extracting valuable insights that enhance health outcomes and elevate patient experiences. Thanks to recent strides in computer science and informatics, AI has swiftly ingrained itself in modern healthcare, playing a pivotal role.
Presently, AI's primary functions in medical settings revolve around clinical decision support and imaging analysis. By quickly accessing relevant patient-related information and research, clinical decision support tools help healthcare providers make smart decisions about treatments, medications, and mental health interventions. In the realm of medical imaging, AI tools scrutinize CT scans, x-rays, MRIs, and other images, detecting anomalies or findings that may elude a human radiologist.
„Artificial intelligence (AI) is defined as “the science and engineering of making intelligent machines”. The application of AI in medicine is anticipated through two main branches – virtual, which involves mathematical algorithms improving learning; physical, which involves the use of robots and medical devices“.![]()
The challenges posed by the COVID-19 pandemic prompted healthcare organizations to embark on trials of AI-supported technologies. These range from algorithms designed to monitor patients to AI-powered tools for screening COVID-19 cases. Although the findings from these tests are still accumulating, and the standards for AI in medicine are evolving, it is evident that opportunities for AI to benefit clinicians, researchers, and patients are on the rise. It is increasingly clear that AI is on track to become an integral component of digital health systems, shaping and fortifying modern medicine.
Applications of AI in Medicine
AI has the potential to revolutionize the field of medicine by expediting research, aiding clinical decision-making, and offering innovative solutions. Here are examples illustrating how AI can be applied:
Disease Detection and Diagnosis
AI can continuously monitor vital signs in critical care patients. It can alert clinicians to elevated risk factors.
Beyond traditional monitoring devices, AI can analyze complex conditions like sepsis and, for example, deliver an accurate predictive model for severe sepsis.
Personalized Disease Treatment
Virtual AI assistants, retaining patient preferences, can offer real-time customized recommendations, providing patients continuous access to tailored healthcare information.
AI in Medical Imaging
Artificial neural networks-powered AI has proven to be just as effective as human radiologists at detecting breast cancer and other conditions.
By streamlining the management of medical images, AI assists clinicians in organizing and presenting relevant patient history, easing the burden of handling vast amounts of imaging data.
Accelerated Drug Development
AI addresses challenges in drug discovery by enhancing drug design and identifying promising combinations, potentially reducing the time and cost associated with developing new medicines.
Benefits of AI in Medicine
„Data science and big data analytics can provide practical insights and aid in the decision-making of strategic decisions concerning the health system. It helps build a comprehensive view of patients, consumers, and clinicians. Data-driven decision-making opens up new possibilities to boost healthcare quality.“![]()
Informed Patient Care
Integrating medical AI into clinician workflows provides valuable context, offering evidence-based insights about treatments and procedures during patient consultations.
Error Reduction
A systemic review of 53 studies found that AI-powered decision support tools improve patient safety by enhancing error detection and drug management.
Reducing Costs of Care
AI offers various opportunities to reduce healthcare costs, such as minimizing medication errors, providing customized virtual health assistance, preventing fraud, and streamlining administrative and clinical workflows.
Increasing Doctor-Patient Engagement
AI ensures around-the-clock support through chatbots, addressing patient queries outside regular hours and potentially triaging questions for further review, enhancing doctor-patient communication.
Providing Contextual Relevance
Deep learning enables AI algorithms to use context to distinguish different types of information, facilitating the identification of relevant details in clinical notes and patient histories.
Transforming Healthcare: The Impact of Artificial Intelligence on Efficiency, Diagnoses, and Patient Care
Artificial Intelligence (AI) is revolutionizing the healthcare industry by leveraging advanced machine learning algorithms, improved data accessibility, cost-effective hardware, and the emergence of 5G technology. This transformative impact is accelerating change, allowing AI and machine learning to swiftly analyze extensive health datasets, including records, clinical studies, and genetic information, surpassing the capabilities of humans.
Operational efficiency and error reduction
One of the key advantages of AI in healthcare lies in enhanced operational efficiency. By streamlining administrative workflows through AI and automation, healthcare professionals can devote more time to direct patient care, reducing the burden of paperwork. Generative AI further aids clinicians in note-taking and content summarization, ensuring comprehensive and thorough medical records.
Virtual nursing assistants, powered by AI, play a pivotal role in providing around-the-clock access to answers through chatbots or apps. This innovation eases routine tasks for clinical staff, allowing them to focus on more critical aspects of patient care.
AI's impact extends to dosage error reduction, where it identifies and mitigates errors in patient self-administration of medications. An example is the use of AI tools to flag errors in insulin pen or inhaler usage, contributing to improved medication adherence.
AI in surgical procedures and fraud prevention
In surgical procedures, AI-enabled robots demonstrate their utility by reducing blood loss, infection risk, and post-surgery pain. These robots navigate around sensitive organs and tissues, making surgeries less invasive and enhancing overall patient outcomes.
Fraud prevention in the healthcare industry is another area where AI shines. By recognizing unusual patterns in insurance claims, AI addresses the staggering $380 billion annual fraud. This reduces medical premiums and out-of-pocket expenses for consumers.
User experience and efficient diagnoses
Improving the user experience is a significant goal of AI in healthcare. Technologies like natural language processing (NLP), predictive analytics, and speech recognition enhance communication between patients and providers, addressing the reported 83% dissatisfaction with poor communication in patient experiences.
According to Harvard's School of Public Health, AI facilitates accurate diagnoses, which could lower treatment costs by up to 50% and improve health outcomes by 40%. AI technologies, including deep learning, enhance breast cancer risk prediction and outperform experienced doctors in recognizing skin cancer.
Health monitoring, preventive care, and data integration
AI supports health monitoring and preventive care by enabling real-time data sharing from health monitors and apps. This facilitates timely alerts and allows doctors to monitor patients' health issues effectively.
AI's role in data integration streamlines the gathering and sharing of healthcare information, aiding providers in managing patient data efficiently. This is evident in diabetes monitoring and drug safety improvements.
Ensuring ethical and regulatory governance is crucial for AI in healthcare. The World Health Organization outlines principles such as
protecting autonomy,
promoting safety and well-being,
ensuring transparency,
fostering accountability,
ensuring equity,
and promoting responsive and sustainable tools.
Looking ahead, the future potential of AI in healthcare includes reducing human error, automating diagnostics, and further enhancing patient care and experience. As AI tools continue to evolve, the healthcare ecosystem stands to benefit from increased automation, streamlined processes, and more compassionate patient care.
Examples from medical research and treatment
#1 Omics and Machine Learning Synergy: Pioneering Predictive Models for Colorectal Cancer Diagnosis and Prognosis
This comprehensive study published in Sensors 2023 navigates the intricate landscape of healthcare by exploring the synergy between omics data and advanced Machine Learning (ML) techniques. The researchers, motivated by the prevalence of colorectal cancer worldwide, focus on the analysis of RNA-seq data from extracellular vesicles (EV) to develop models for predicting colon cancer and classifying its stages.
In their quest, the team employs a diverse array of ML and Deep Learning (DL) classifiers, ranging from k-Nearest Neighbor and Random Forest to sophisticated models like 1-D CNN and Bidirectional LSTM. The results not only showcase the promising performance of these models but also unveil the superiority of DL algorithms in terms of accuracy, shedding light on their potential in revolutionizing disease prediction and classification.
What sets this study apart is its emphasis on the practical applicability of the proposed approach. Beyond the realm of theoretical research, the authors envision a role for their models in the real-world medical landscape. Acting as a complementary tool for medical professionals, these models could provide valuable second opinions, particularly in cases where diagnoses might be challenging or disagreements among healthcare practitioners arise.
The potential applications extend to both diagnosis and prognosis, where the approach could significantly contribute to treatment planning. By analyzing patients' exRNA profiles, the models offer insights that can support medical doctors in reaching consensus on diagnoses and determining the stage of cancer. This not only accelerates treatment planning but also enhances the chances of successful recovery and improves the overall quality of life for patients.
Furthermore, the study envisions broader applicability, suggesting that similar approaches could be employed for other types of cancer. ML and DL models can be improved and retrained by getting exRNA profiles from healthy people and patients with certain cancers. This makes them more reliable and able to adapt to changing healthcare situations.
In the context of the ongoing COVID-19 pandemic, the study's emphasis on easing the workload on medical professionals resonates strongly. The proposed approach, if implemented, could offer consistent decision support, backed by artificial intelligence, potentially alleviating the time constraints on medical doctors and allowing them to focus on critical patient care aspects.
In conclusion, this study not only looks into the technical aspects of combining omics data with machine learning methods, but it also hopes to have a real effect on healthcare, where the proposed approach could become a useful tool for doctors in diagnosing and predicting the outcomes of complicated diseases like colon cancer.
#2 Revolutionizing Clinical Trials: A Roadmap to Transformative Patient-Centric Research and Global Drug Development
An article published in Nature Magazine shows the evolving landscape of evidence-based medicine, driven by advancements in wearable technologies, data science, and machine learning. The author envisions a future of clinical trials marked by innovation and patient-centricity. Despite remarkable progress in scientific understanding and technology, the translation of these advancements into clinical applications lags in various medical fields. The COVID-19 pandemic, while exposing systemic limitations in clinical trials, also catalyzed positive changes, fostering a shift towards patient-centric evidence generation.
The need for unconventional strategies
The article underscores the need for unconventional strategies in trial design, emphasizing the limitations of traditional randomized controlled trials (RCTs). It highlights the progress made in 'master' protocols, such as umbrella studies, basket trials, platform studies, and master observational trials (MOTs), particularly in oncology. Biomarker development is identified as a crucial component for expediting drug development across diverse medical disciplines.
Additionally, the article explores the positive impact of the pandemic on reshaping clinical trial conduct, leading to more patient-centric, decentralized, and technologically driven approaches. The integration of artificial intelligence is proposed to enhance the patient experience and streamline trial processes. The overarching message advocates for a paradigm shift in clinical research models, urging the adoption of innovative, patient-centric strategies and the incorporation of emerging technologies to propel advancements in medicine.
The article clarifies the complex network of individuals who contribute to patient recruitment in clinical trials while highlighting the crucial part that clinical investigators, doctors, and other healthcare professionals play. While major academic centers excel in efficiently managing trial portfolios and patient screening, most trial sites face challenges, including staffing constraints.
The clinical trial research enterprise, as depicted, involves a complex interplay among patients, academic centers, industry sponsors, regulatory agencies, and more. A pressing need for a digital overhaul is highlighted, pointing out the prevalence of outdated practices such as protocol binders and pen-and-paper diaries. The integration of blockchain technology is proposed to enhance trust and streamline the clinical trial management system.
Patient participation is deemed crucial, calling for improved accessibility to trials and the inclusion of clinical trial options in treatment decisions. The article advocates for clinical trial training as part of medical education, ensuring a diverse pool of trained investigators for robust clinical research.
Addressing the urgency of drug development timelines, the article draws attention to the unprecedented speed of COVID-19 vaccine and treatment development, emphasizing the need to apply such 'lightspeed approaches' to various therapeutic areas.
Globalizing drug development and achieving harmonization among regulatory authorities are recognized as vital for addressing inequities in access to medicines. The potential of transportability analyses in evidence generation across diverse populations is explored.
The future of clinical trials
The article talks about how clinical research is changing, with a focus on how molecular biology and laws supporting orphan disease therapeutics have made it easier to study rare diseases. Notable examples include accelerated approval based on real-world data (RWD) for rare disorders.
The role of N-of-1 trials in studying rare diseases is highlighted, with a glimpse into the emerging concept of medical analog patient-specific 'digital twins.' The article addresses the gap between conventional clinical trials and the real-world population, advocating for the integration of RWD and real-world evidence (RWE).
Synthetic control arms are proposed as innovative approaches in drug development, particularly for rare diseases, with examples showcasing their effectiveness. Challenges and potential future uses are discussed, paving the way for a paradigm shift in evidence generation.
The article touches upon the complexities of pediatric clinical trials, emphasizing the need for creative methods, leveraging RWD, and incentivizing pediatric therapeutic development through legislative acts.
In reimagining the future of clinical trials, the integration of artificial intelligence (AI) is highlighted. While AI has made significant strides in clinical care, its application in clinical trial research is slower, with the article advocating for collaborative AI-human scenarios. Standardized reporting guidelines for AI in clinical trials are introduced.
The article concludes by stressing the imperative need for a fundamental transformation in clinical trial design, conduct, and reporting. There is a call for ongoing disruptive innovation, breaking down silos, and integrating healthcare systems into intuitive real-world evidence generation. The COVID-19 pandemic is seen as a catalyst for better clinical care and trial efficiency. The vision includes every major disease having a 'Moonshot' program and every rare disease having an 'Operation Warp Speed' for sustainable progress in personalized medicine.
Scientists have looked into how epigenetics affects migraines and found that genetics, environmental factors, and epigenetic processes such as DNA methylation and histone modifications all work together. Genes like RAMP1, SH2D5, NPTX2, and GRID2 were found to have abnormal DNA methylation patterns in people who get migraines. Epigenetics could lead to more personalized treatment options, with DNMT inhibitors and miRNA-based therapies being two possible targets. Lifestyle factors, including stress and diet, influence epigenetic changes in migraine-related genes. Epigenetic research offers hope for understanding and treating migraines, with the promise of improved therapies in the future.
#3 Decoding Migraine Mysteries: Epigenetic Insights and Personalized Treatments Unveiled Through Data-driven Discoveries
In their exploration of the mysteries of migraines, researchers have delved into the intricate world of epigenetics, shedding light on the complex interplay between genetic predisposition and environmental influences. Migraine, a debilitating neurological disorder, involves abnormal DNA methylation patterns in specific genes like RAMP1, SH2D5, NPTX2, and GRID2. This abnormal methylation can influence gene expression and contribute to the severity of migraine attacks.
Epigenetic mechanisms, including histone modifications and microRNAs (miRNAs), play crucial roles in migraine pathogenesis. Histone modifications, such as H3K27me3, regulate microglial cell activation, linking epigenetics to the inflammatory processes underlying migraines. MiRNAs, like miR-27b and miR-181a, demonstrate altered expression in migraine patients, impacting processes related to inflammation, pain perception, and neuronal excitability.
The potential for personalized migraine treatments lies in targeting epigenetic modifications. DNA methyltransferases (DNMTs) and histone deacetylases (HDACs) inhibitors show promise in preclinical studies, offering avenues for pain reduction in migraine. Additionally, miRNA-based therapies present opportunities to modulate gene expression and alleviate symptoms.
Beyond genetics, environmental factors like stress, diet, and lifestyle choices influence epigenetic changes in migraine-related genes. Stress, in particular, induces DNA methylation alterations in stress response genes. Dietary factors, including vitamins B6, B12, and folate, impact DNA methylation patterns in genes associated with migraines.
In conclusion, epigenetic research stands as a beacon of hope in the realm of migraine studies. It provides a holistic understanding of the disorder's molecular basis and opens doors to personalized and effective treatment strategies. As researchers continue to unravel the secrets of epigenetic regulation, the prospect of offering relief and hope to those suffering from migraines draws closer.
We recently addressed the study in another blog post. You can find the summary here.
The Intersection of AI and Medicine: A Glimpse into the Future
In the ever-evolving landscape of healthcare, artificial intelligence (AI) stands as a transformative force, reshaping how we approach diagnostics, treatments, and patient care. We are witnessing a paradigm shift as we learn more about how AI and medicine can work together to improve efficiency, make accurate diagnoses, and make patient experiences more important.
AI's pivotal roles
At the forefront of AI's impact in medicine are clinical decision support and medical imaging analysis. These functions empower healthcare providers with rapid access to relevant patient data, facilitating informed decisions on treatments, medications, and mental health interventions. In the realm of medical imaging, AI tools emerge as meticulous observers, scrutinizing CT scans, x-rays, and MRIs to unveil anomalies that might elude human radiologists.
Applications across the medical spectrum
The applications of AI in medicine are vast and varied. Disease detection and diagnosis witness a revolution, with AI continuously monitoring vital signs and alerting clinicians to elevated risk factors. Virtual AI assistants, retaining patient preferences, offer real-time customized recommendations, ushering in a new era of personalized disease treatment.
In the realm of medical imaging, artificial neural networks empower AI to rival human radiologists in detecting conditions like breast cancer. Clinical trials, often cumbersome in their processes, find a streamlined ally in AI, expediting tasks like assigning medical codes and accelerating drug development.
Benefits for a healthier future
The benefits of AI in medicine are manifold. Informed patient care becomes a reality as medical AI integrates seamlessly into clinician workflows, providing evidence-based insights during consultations. Error reduction takes center stage, enhancing patient safety through improved error detection and drug management.
AI not only promises to reduce healthcare costs but also fosters increased doctor-patient engagement. Through chatbots and continuous support, AI addresses patient queries outside regular hours, bridging communication gaps and fostering a more connected healthcare experience.
Transformative impact on efficiency, diagnoses, and patient care
Advanced machine learning algorithms, improved data accessibility, cost-effective hardware, and the emergence of 5G technology have all contributed to AI's transformative impact on the healthcare sector. From operational efficiency and error reduction to virtual nursing assistants and enhanced diagnoses, AI stands as a catalyst for positive change.
The integration of AI in surgical procedures and fraud prevention showcases its versatility, making surgeries less invasive and addressing systemic issues in the healthcare industry. User experience is elevated through technologies like natural language processing, predictive analytics, and speech recognition, enhancing communication between patients and providers.
Looking ahead
As we peer into the future, the potential of AI in healthcare continues to expand. With a focus on reducing human error, automating diagnostics, and enhancing patient care, AI's trajectory is clear. Ongoing innovations exemplify the ongoing integration of AI to provide quick and efficient answers, contributing to improved accessibility and efficiency in healthcare services.
The path ahead involves navigating ethical and regulatory considerations, ensuring AI in healthcare adheres to principles of autonomy, safety, transparency, accountability, equity, and sustainability outlined by the World Health Organization. As AI tools evolve, the healthcare ecosystem is poised to benefit from
increased automation,
streamlined processes,
and a more compassionate approach to patient care.
The future of medicine is being shaped by the collaboration of human expertise and artificial intelligence, promising a healthier and more connected world.