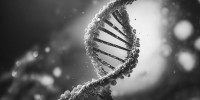
The Future of Genomics Data Analysis with AI
Discover how the integration of Artificial Intelligence is revolutionizing genomics, unlocking new possibilities in precision medicine, drug discovery, and early disease detection, while addressing challenges of data privacy and model transparency. Explore the future of healthcare innovation as AI and genomics converge to transform our understanding of human biology.
AI and Genomics: Pioneering a New Era in Precision Medicine and Genetic Insights
At a Glance
Market Growth: The AI in genomics market is expected to grow from $0.5 billion in 2023 to $2.0 billion by 2028, driven by the need for streamlined processes and enhanced precision medicine.
Precision Medicine: AI enables personalized treatment plans by integrating and analyzing diverse data types, predicting individual responses to therapies.
Drug Discovery: AI accelerates drug discovery by identifying potential therapeutic targets and simulating drug interactions, reducing costs and increasing success rates.
Early Detection: AI identifies biomarkers and genetic patterns for early disease detection, facilitating proactive health interventions.
Functional Genomics: AI predicts the effects of non-coding genetic variants, aiding in the understanding of complex biological networks.
CRISPR and AI: AI enhances genome editing precision, designing optimal guide RNAs for CRISPR systems, and optimizing base and prime editing.
Explainable AI (XAI): XAI provides transparency in AI models, crucial for understanding genomic regions and disease mechanisms, especially in cancer research.
Enhancers in Cancer: Understanding enhancer regions helps in identifying disease-causing mutations, crucial for cancer genomics and targeted therapies.
Challenges: Key challenges include data privacy, ethical considerations, and the need for transparent, interpretable AI models.
Introduction
The integration of Artificial Intelligence (AI) into genomics marks a revolutionary era in scientific discovery and healthcare innovation. As the genomics landscape evolves, AI's role in transforming data analysis capabilities and expanding the horizons of genetic research becomes increasingly critical. This synergy promises to unlock unprecedented insights into disease mechanisms, drug development, and personalized medicine.
The Genomics Data Explosion and AI's Role
The rapid advancement in sequencing technologies has resulted in an unparalleled influx of genomics data. This vast data landscape, while rich with potential insights, presents significant analytical challenges. Traditional bioinformatics approaches often fall short in handling the complexity and volume of modern genomic datasets. AI, particularly through machine learning (ML) and deep learning (DL), offers a powerful toolkit for addressing these challenges.
According to MnM Healthcare Industry, the AI in genomics market is projected to grow from $0.5 billion in 2023 to $2.0 billion by 2028, propelled by the need to streamline processes, reduce costs, and enhance precision medicine. This growth is driven by AI's ability to analyze complex genomic patterns and predict disease risks with greater accuracy.
Transformative Applications of AI in Genomics
Precision Medicine
AI's ability to integrate and analyze diverse data types—genomic, clinical, and phenotypic—facilitates the development of personalized medicine. Algorithms can predict individual responses to treatments, enabling healthcare providers to tailor interventions based on a patient’s genetic profile. This precision medicine approach is becoming a cornerstone of modern healthcare, promising improved patient outcomes and reduced adverse effects.
Drug Discovery and Development
AI can significantly expedite drug discovery by identifying potential therapeutic targets from genomic data. As noted by MnM Healthcare Industry, AI's application in drug discovery reduces costs and increases the success rate of clinical trials. By simulating drug interactions and responses, AI-driven platforms can streamline the development pipeline, leading to faster availability of new treatments.
Early Disease Detection
AI algorithms are adept at identifying biomarkers and genetic patterns associated with diseases, facilitating early detection and prevention strategies. By targeting high-risk populations, AI can help implement proactive health interventions, ultimately leading to better health outcomes and cost savings for healthcare systems.
Functional Genomics
Understanding the functional implications of genetic variants is crucial for translating genomic insights into clinical practice. AI models can predict the effects of non-coding variants on gene regulation, aiding in the elucidation of complex biological networks and mechanisms.
AI and Genome Editing: Unlocking New Possibilities
The integration of AI into genome editing technologies, such as CRISPR, is unlocking new possibilities for precision medicine and therapeutic interventions. As Dixit et al. (2024) explain in their review published in Frontiers in Bioengineering and Biotechnology, AI enhances the precision and efficiency of genome editing by designing optimal guide RNAs (gRNAs) for CRISPR-Cas systems. Tools like DeepCRISPR and CRISTA utilize AI to predict gRNA efficiency, taking into account genomic context and potential off-target effects.
AI-driven models also optimize advanced genome editing techniques, including base editing and prime editing, facilitating precise and programmable changes to DNA sequences. This synergy between AI and genome editing is pivotal in developing personalized treatments based on genetic profiles, enabling targeted interventions for diseases such as cancer, diabetes, and Alzheimer's.
Challenges and Considerations
Despite its potential, the integration of AI in genomics is not without challenges. Data privacy and security remain paramount concerns, especially given the sensitive nature of genetic information. As highlighted by Chris Middleton in diginomica, the need for equitable AI models that perform well across diverse populations is critical to ensuring fair and accurate healthcare outcomes.
Moreover, the complexity of biological data demands that AI models are interpretable and transparent. This requires collaboration between computational scientists and biologists to ensure that AI-generated insights are biologically meaningful and actionable. The ethical and regulatory considerations of deploying AI in gene editing, as discussed by Dixit et al. (2024) as well as many other researchers, also necessitate robust frameworks to ensure safe and responsible application.
On our own behalf: We know that medical data analytics can be time-consuming and complicated. But the perfect solution is coming soon: Be ready for our game-changing analytics platform. If you want to become one of our first alpha-testers with early access, please send us an email to info@aimed-analytics.com or get in touch via our contact-button on our website.
The Role of Explainable AI (XAI) in Genomics
The need for transparency and interpretability in AI models is increasingly recognized, particularly in sensitive fields like genomics and healthcare. In their review published in Discover Artificial Intelligence, Maqsood et al. (2024) emphasize the importance of Explainable Artificial Intelligence (XAI) in providing transparency and trust in AI's decision-making processes. XAI aims to make AI models understandable to humans by explaining how predictions are made and identifying the underlying data features that drive these decisions.
In genomics, XAI can help uncover the molecular principles underlying disease states by enhancing the understanding of genomic regions, particularly non-coding regulatory regions like enhancers. Maqsood et al. note:
The understanding of genomic regions, specifically the non-coding regulatory region of genomes (i.e., enhancers), can help uncover underlying molecular principles of disease states, in particular cancer in humans.![]()
This understanding is crucial for identifying potential therapeutic targets and improving the development of personalized medicine.
Enhancers and Cancer: A Case Study
Enhancers, as non-regulatory elements, are responsible for controlling the transcription of one or more genes. The characterization and identification of these regions are vital in understanding human disease causation and aiding in the development of novel drugs. Maqsood et al. (2024) also highlight the complexity of enhancers:
The position of enhancers is variable and relative to their target and can occur downstream, upstream, or within the introns of a gene. Enhancers may make 3D contact with promoters to achieve regulation of distal genes. Furthermore, there is no specific motif or code for enhancers, and they may only be active in specific temporal, environmental, and spatial conditions. These characteristics of enhancers make it difficult to identify and annotate the enhancers.![]()
AI, particularly through XAI, can play a pivotal role in predicting and understanding enhancer regions. This approach allows researchers to identify disease-causing mutations and develop targeted therapies, thereby advancing cancer genomics and personalized medicine.
The Future Outlook
The future of genomics data analysis with AI is one of immense promise and potential. Interdisciplinary collaboration will be essential to addressing the ethical, legal, and social implications of AI-driven genomics. As AI technologies continue to evolve, they will play an increasingly central role in advancing precision medicine, understanding complex genetic diseases, and developing novel therapies.
The insights from Genomics England's efforts, as shared by Dr. Rich Scott, emphasize the importance of integrating AI with national healthcare systems to manage and utilize genomic data effectively. This approach not only enhances the efficiency of data analysis but also ensures that insights are accessible for research and clinical applications.
Conclusion
The integration of AI in genomics represents a transformative shift in how we understand and utilize genetic information. By overcoming the limitations of traditional methods, AI offers a pathway to more accurate, efficient, and personalized genomic analyses. As we continue to explore the possibilities of this rapidly evolving field, AI's role in genomics promises to drive significant advancements in healthcare, leading to improved patient outcomes and a deeper understanding of human biology. The future of genomics, empowered by AI, stands at the cusp of a new era of discovery and innovation.