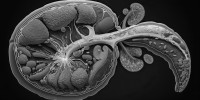
Uncovering Early Signs of Pancreatic Cancer via Transcriptomics
Could understanding the early changes in pancreatic lesions be the key to saving lives? New research is shedding light on the early signs of this challenging disease, offering hope for earlier detection and better treatment options. By utilizing cutting-edge techniques, scientists are uncovering crucial insights into how pancreatic lesions evolve into cancer, potentially transforming our approach to this deadly illness.
Uncovering Early Signs of Pancreatic Cancer via Transcriptomics
At a Glance
Early Detection and Markers
Early Signs of Pancreatic Cancer: Researchers have identified new markers in early-stage pancreatic lesions, known as pancreatic intraepithelial neoplasias (PanINs), that can eventually turn into pancreatic cancer. This breakthrough was achieved using cutting-edge techniques in spatial transcriptomics and machine learning.
Combining Spatial Transcriptomics with AI: By combining spatial transcriptomics with machine learning tools like CODA and ProjectR, scientists have mapped out how genes behave and where cells are located within these early lesions, offering crucial insights into how pancreatic cancer develops from its earliest stages.
Key Role of Fibroblasts: Certain types of fibroblasts—cells known to support tumor growth—were identified near PanINs, suggesting they’re already influencing the cancerous environment well before full-blown pancreatic cancer develops.
Immune Response and Treatment Implications
Immune System Suppression Starts Early: High-grade PanINs were found to suppress inflammation and increase cell growth, creating an environment that allows cancer to progress more easily. This immunosuppressive shift happens even before invasive cancer takes hold.
Tools for Early Detection and Treatment: This study is making its data and analysis methods available to the broader research community, helping pave the way for new tests and treatments that could detect pancreatic cancer earlier than ever.
Importance of Spatial Analysis: Understanding the physical layout of cells, including immune cells and fibroblasts, around these early lesions is critical. Knowing which cells are close to each other and how they interact can help refine strategies to treat or even prevent pancreatic cancer.
Advancements in Transcriptomics, Data Analysis, and Machine Learning in Cancer Research: Focusing on Pancreatic Cancer
Transcriptomics—the study of RNA molecules and their expression in cells—is a central component of cancer research, particularly in understanding complex cancers like pancreatic cancer. By analyzing which genes are active or inactive, transcriptomics provides insights into cellular behavior, tissue environments, and disease progression.
With recent advancements in spatial transcriptomics and machine learning, researchers are making strides in uncovering molecular mechanisms and biomarkers that are critical to early detection and treatment response, especially in pancreatic ductal adenocarcinoma (PDAC).
Understanding the Landscape of Transcriptomics in Cancer Research
Cancer is fundamentally driven by genetic and transcriptomic changes, making transcriptomics invaluable for mapping out gene expression profiles associated with different cancer stages.
Techniques such as bulk RNA sequencing and single-cell sequencing allow scientists to identify expression patterns in both cancerous cells and those in the tumor microenvironment. However, these methods have historically struggled to capture the spatial relationships between cells within tissues—an important aspect for understanding how tumors interact with surrounding cells to promote cancer progression.
Enter spatial transcriptomics, a revolutionary technique that integrates transcriptomic data with spatial information about cell locations within a tissue sample. This advancement is proving crucial in the study of cancers like PDAC, where the intricacies of the microenvironment can provide new insights into immune responses, cellular behaviors, and potential therapeutic targets.
Insights from Pancreatic Cancer Research: A Focus on PanIN Lesions
Recent research led by a team at Johns Hopkins University, headed by Dr. Luciane Kagohara, has unveiled significant markers in the early stages of PDAC. Pancreatic intraepithelial neoplasias (PanINs) are precancerous lesions that can arise years before the onset of PDAC. However, their small size (often less than 1mm) makes them nearly impossible to detect with traditional imaging methods.
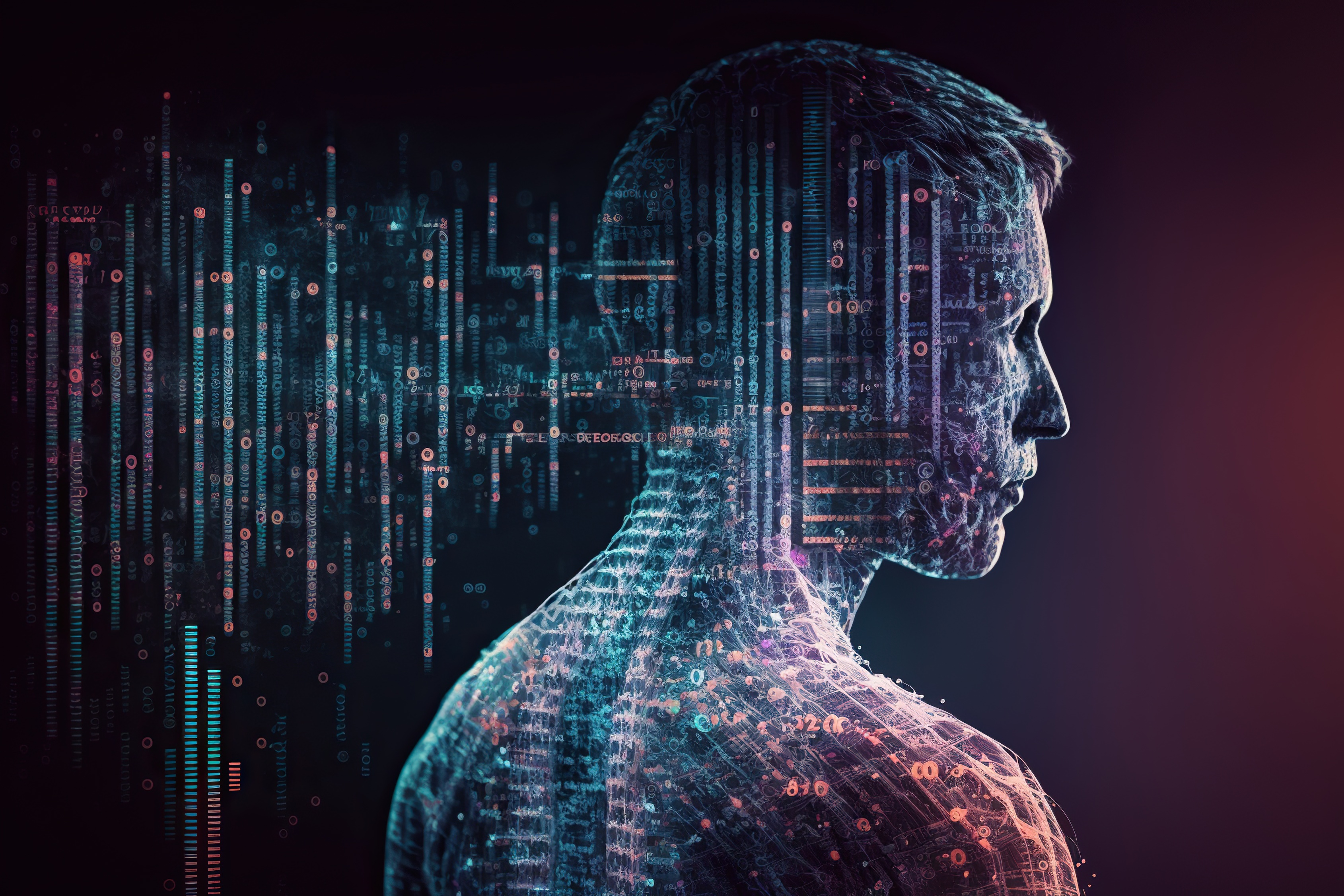
By employing spatial transcriptomics and machine learning, Dr. Kagohara’s team successfully mapped gene expression and cellular distribution within PanINs, revealing how certain cells contribute to an environment that supports cancer development even in its earliest stages.
The researchers utilized a three-pronged analytical approach to track changes in gene expression across various grades of PanIN lesions. Their findings indicate that high-grade PanINs already exhibit certain molecular characteristics typical of invasive PDAC, such as the presence of specific types of cancer-associated fibroblasts (CAFs). These fibroblasts, known to influence tumor growth and immune response, were found close to PanINs, highlighting their potential role in the early stages of pancreatic cancer development.
The Role of Machine Learning in Analyzing Transcriptomic Data
Analyzing the complex data generated by spatial transcriptomics requires advanced computational tools, and this is where machine learning comes into play. The Johns Hopkins team adapted a machine-learning method called CODA to automatically identify and annotate different cell types within the pancreatic microenvironment. Additionally, they used ProjectR, a transfer-learning algorithm, to explore how inflammatory patterns associated with CAFs change during PDAC progression.
Machine learning enables researchers to handle high-dimensional data more effectively, making it easier to extract meaningful insights from vast datasets. As Dr. Luciane Kagohara, senior study co-author and assistant professor of oncology at the Johns Hopkins University School of Medicine noted:
With the right tools, you can enhance the results that you can extract from these novel technologies.![]()
This ability to analyze complex data not only improves our understanding of pancreatic cancer but also helps researchers design better-targeted therapies.
Uncovering Early Markers of Pancreatic Cancer Progression
One of the standout discoveries from this research is the way cellular behavior shifts as PanINs progress to higher grades of malignancy. As Mark Fuerst reported in Oncology Times, Dr. Kagohara observed that:
When we looked at the progression of normal to high-grade PanIN lesions, we found that cell proliferation gradually increases while inflammatory signaling decreases, which can be important to understand the intrinsic low immunogenicity of these tumors.![]()
This suggests that even at the precancerous stage, PanINs are creating an environment that suppresses immune responses, which could facilitate the cancer’s eventual invasion.
Moreover, the presence of specific CAFs at such an early stage marks a significant finding. While previous animal studies hinted at the role of CAFs in PDAC progression, this research provides one of the first confirmations of their presence in human PanIN lesions. This insight could be pivotal in developing preventive strategies and understanding how to combat the cancer before it fully develops.
Open-Source Data and the Future of Pancreatic Cancer Research
In a spirit of collaboration, the team has made their data and methodologies available to the research community. By sharing these resources, they aim to inspire further exploration into pancreatic cancer progression and develop new tools for early detection. Dr. Kagohara emphasizes that as researchers continue to generate more data on PanINs, the goal is to identify distinct molecular signatures that could lead to the creation of effective early detection tests.
This open-source approach reflects a growing trend in cancer research, where collaboration and data sharing accelerate the pace of discovery. As technologies in transcriptomics advance and machine learning becomes more sophisticated in handling spatial and multi-omics data, the potential for early cancer detection and tailored therapies will continue to expand.
Conclusion
The integration of transcriptomics, spatial data analysis, and machine learning is revolutionizing our understanding of cancer biology, with significant implications for pancreatic cancer research. The work by Dr. Kagohara and her colleagues demonstrates the power of these tools in identifying early molecular markers and elucidating the spatial dynamics of cancer-associated cells. With pancreatic cancer often diagnosed too late for effective treatment, the ability to detect changes in PanINs offers hope for earlier diagnosis and intervention.
As Dr. Kagohara pointed out, understanding the proximity of immune cells to tumors and the barriers created by other cell types is crucial for effective treatment strategies. The ongoing advancements in transcriptomics and data analysis could ultimately transform how we approach cancer treatment, providing new pathways for early diagnosis, personalized therapies, and improved outcomes for patients facing one of the most challenging cancers.