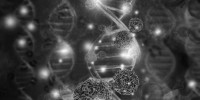
Revolutionizing Cancer Treatment with Deep Learning
Discover how cutting-edge deep learning models are revolutionizing cancer treatment by predicting drug responses and uncovering new therapeutic possibilities through drug repurposing. Dive into the latest advancements in precision medicine today!
Harnessing Deep Learning for Precision Cancer Treatment
Cancer remains one of the most formidable challenges in modern medicine. While surgery, chemotherapy, and targeted drug therapies offer hope, they also come with significant drawbacks. The development of new cancer drugs is often a costly and time-consuming endeavor.
However, a recent research article has introduced a promising solution utilizing deep learning to predict cancer drug response and facilitate drug repurposing.
At a Glance
Approach: Deep learning model using multi-omics data, drug descriptors, and drug fingerprints.
Datasets Used: GDSC, CTRP, CCLE.
Key Techniques: Autoencoders for dimensionality reduction, Multi-Task Learning (MTL) model.
Performance: Achieved AUPRC of 0.99 in internal testing and 0.72 in cross-dataset evaluation.
Potential: Significant advancements in precision medicine and drug repurposing.
Introduction
Cancer treatment has long been a complex and multifaceted challenge. Traditional methods such as chemotherapy and surgery, while effective, come with serious side effects and high costs.
Drug repurposing—using existing drugs for new therapeutic purposes—emerges as an attractive alternative. Computational techniques, particularly deep learning, have shown remarkable promise in this domain.
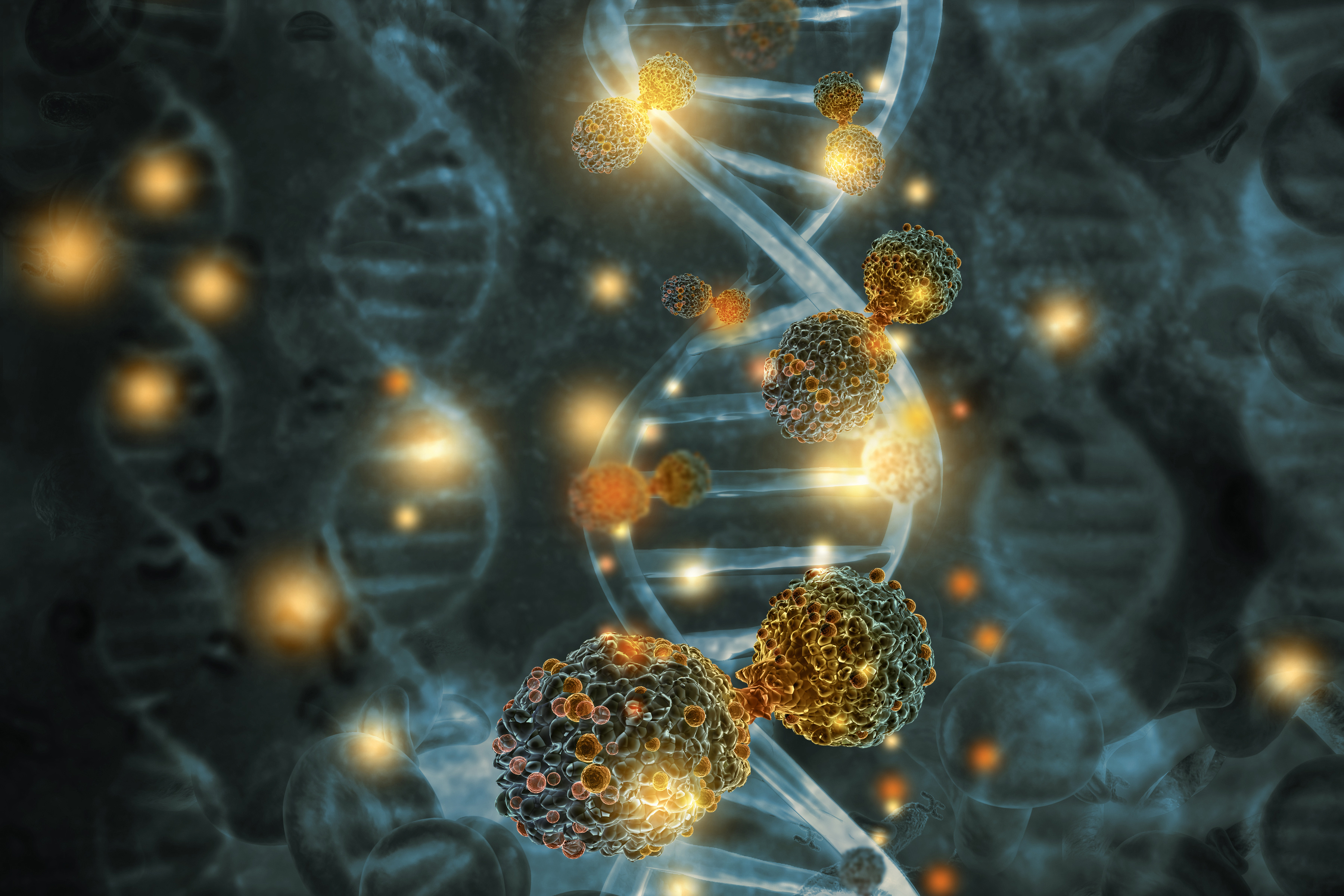
The Promise of Deep Learning
Deep learning excels at identifying complex patterns within large datasets, making it an invaluable tool for predicting how cancer cells will respond to various drugs. This article specifically highlights the advantages of deep learning over classical machine learning methods, noting:
Deep learning’s ability to discern intricate patterns in large datasets makes it one of the most powerful tools for drug response prediction.![]()
Methodology
So, the researchers developed a deep learning model that leverages multi-omics data, drug descriptors, and drug fingerprints to predict cancer drug responses. The multi-omics data was made less complicated using autoencoders. This data was then linked to Multi-Layer Perceptrons (MLPs) to create a strong prediction model.
Key Datasets
GDSC (Genomics of Drug Sensitivity in Cancer)
CTRP (Cancer Therapeutics Response Portal)
CCLE (Cancer Cell Line Encyclopedia)
These datasets were meticulously curated and preprocessed to ensure the integration of critical multi-omics information and drug response data.
Experimental Results
The study conducted seven comprehensive experiments to test the model's performance and generalization capabilities:
Ablation Study: Demonstrated the crucial role of autoencoders in the model’s performance, achieving an AUPRC of 0.91 with both Cell Line and Drug Autoencoders.
Single Omic vs. Multi-Omic Integration: Showed that integrating multiple omics data types significantly improved model performance.
Mixed Dataset Methodology: The model, trained on GDSC and CTRP, outperformed existing models like DeepDRK.
Cross-Dataset Evaluation: Training on GDSC and CTRP together with testing on CCLE showed that the model was good at generalization, with an AUPRC of 0.87.
Internal Consistency: Evaluated through 5-fold cross-validation on the GDSC dataset, the model achieved an impressive AUPRC of 0.98.
Drug Repurposing
One of the exciting applications of this model is drug repurposing. After training on the combined GDSC and CTRP datasets, the model was tested on breast cancer cell lines. It identified several drugs, including some not previously recognized for breast cancer treatment, showcasing the model’s potential to uncover novel therapeutic options.
Conclusion and Future Directions
This deep learning model has showcased its potential to revolutionize cancer treatment by improving drug response predictions and enabling drug repurposing. The article . concludes:
Using this model, we could assess drug responses and explore drug repurposing, leading to the discovery of novel cancer drugs.![]()
Future research aims to enhance the model's denoising capabilities, incorporate additional omics data, and further validate its clinical relevance.
Final Thoughts
The integration of deep learning into cancer treatment represents a significant step forward in precision medicine. By leveraging advanced computational techniques, researchers can now predict drug responses with remarkable accuracy, paving the way for more effective and personalized cancer therapies.