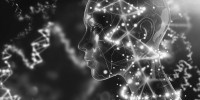
AI in Pharmaceutical Research: A Transformative Revolution
Artificial Intelligence (AI) has become a pivotal force in pharmaceutical research, revolutionizing the development of new therapies and the optimization of existing ones. With the integration of machine learning (ML) and deep learning (DL) technologies, AI is accelerating drug discovery, streamlining processes, and reducing the time and cost traditionally associated with developing new drugs.
Let's explore the growing impact of AI on pharmaceutical research and its key applications, challenges and future potential.
Artificial Intelligence in Pharmaceutical Research: A Transformative Revolution
At a Glance
AI in pharmaceutical research is transforming drug discovery by enhancing target identification, speeding up compound screening, and predicting drug toxicity.
By analyzing large datasets such as genomics, proteomics, and clinical data, AI helps researchers design more effective, personalized treatments.
Despite challenges related to data quality and model generalizability, AI offers exciting opportunities to reduce costs, improve patient outcomes, and accelerate drug development.
AI’s Role in Revolutionizing Pharmaceutical Research
The integration of AI into pharmaceutical research has dramatically changed how researchers approach drug development or biomedical data analysis. As Lu et al. (2023) state in their review:
The processing and analysis of such large-scale omics data (genomics, transcriptomics, proteomics, metabolomics, etc.) have been revolutionary to biology, medicine, and pharmacology, especially in facilitating researchers’ understanding of complex biological systems and processes.![]()
By processing vast amounts of data, AI enables the identification of drug targets, optimization of therapeutic candidates, and the prediction of their interactions, all while reducing the time and resources required in traditional research methods.
From target identification to drug repurposing, AI is making a significant impact throughout the entire pharmaceutical research process. By analyzing large datasets—ranging from genomic to proteomic and clinical data—AI helps researchers identify new drug targets, screen chemical libraries, and predict the efficacy and safety of compounds before moving to experimental stages.
Key AI Applications in Pharmaceutical Research
1. Target Identification
AI plays a crucial role in target identification, allowing researchers to uncover disease-associated targets and molecular pathways that could be modulated to treat specific conditions. By analyzing genomic and proteomic data, AI helps pinpoint key biological targets more efficiently, enabling researchers to bypass many of the limitations of traditional methods.
2. Virtual Screening
In virtual screening, AI models simulate chemical interactions to predict how well a potential drug will bind to its target. This in silico technique enables the rapid screening of large chemical libraries, helping researchers identify promising compounds for experimental testing, thus saving both time and money in the early stages of pharmaceutical research.
3. Drug Repurposing
AI also aids in drug repurposing, where existing drugs are analyzed to find new therapeutic uses. By exploring relationships between approved drugs and different diseases, AI identifies new indications for existing drugs, accelerating the development process and reducing costs in pharmaceutical research.
4. Toxicity Prediction
One of AI’s most important roles in pharmaceutical research is predicting the toxicity of potential drug candidates. Through the analysis of chemical structures, AI can anticipate harmful side effects, allowing researchers to prioritize safer drug candidates and reduce the risks of clinical trial failures.
5. De Novo Drug Design
AI-driven generative models, such as reinforcement learning, can design entirely new drug-like molecules. By analyzing data from existing chemical libraries, AI expands the chemical space and proposes novel compounds, offering new opportunities for drug discovery in pharmaceutical research.
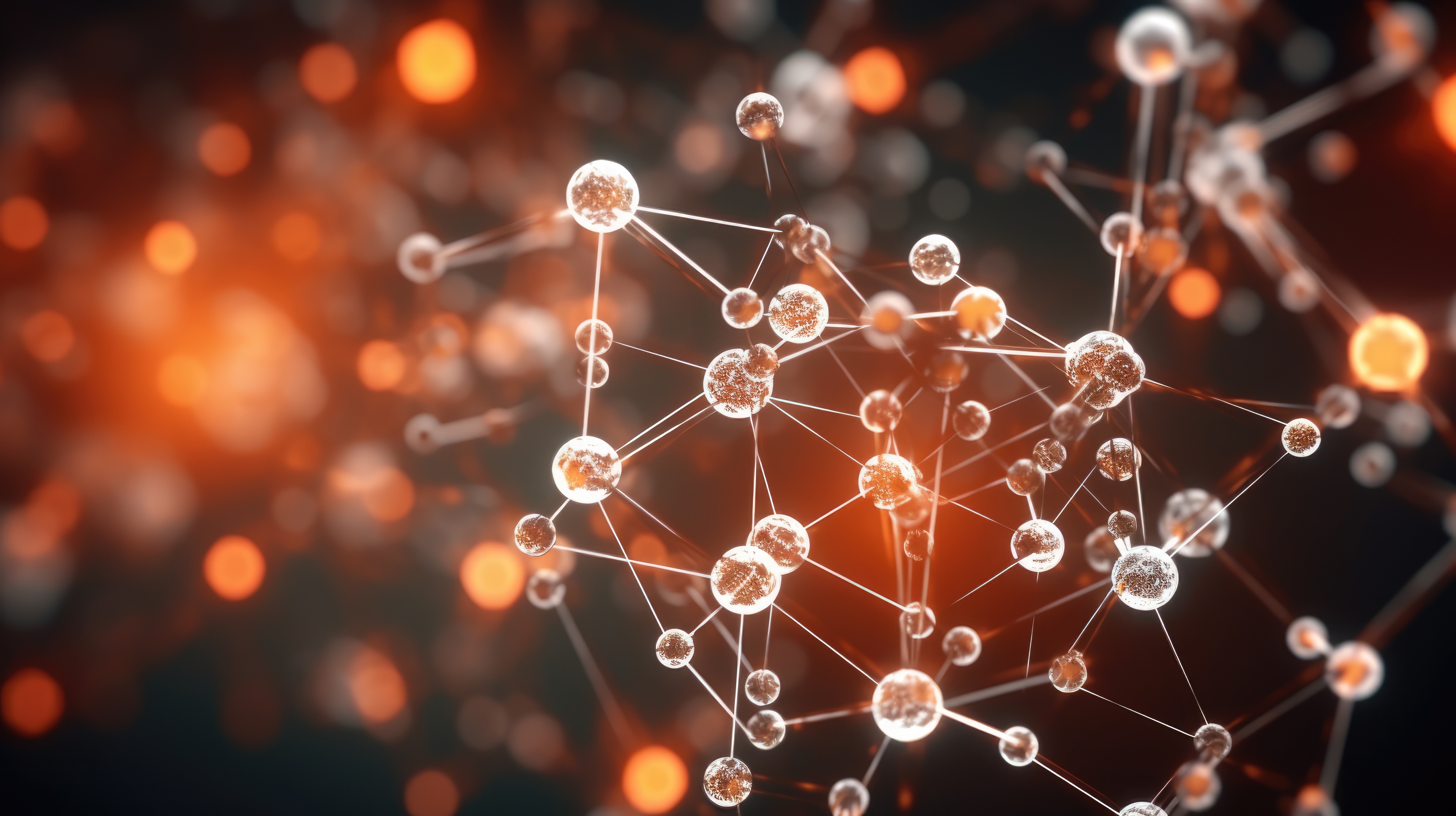
Optimizing Drug Candidates with AI
Once drug candidates are identified, AI algorithms help optimize their efficacy, safety, and pharmacokinetics. By analyzing factors such as molecular structure, AI refines drug candidates, making them more effective and minimizing adverse effects. Vora et al. (2023) emphasize, and many other researchers would certainly agree, that:
AI algorithms can analyze and optimize drug candidates by considering various factors, including efficacy, safety, and pharmacokinetics.![]()
This enables them to ensure the best possible results for pharmaceutical research.
Challenges in AI-Driven Pharmaceutical Research
Although AI offers many advantages, several challenges still need to be addressed for its full potential in pharmaceutical research to be realized.
1. Data Quality and Availability
The effectiveness of AI in pharmaceutical research is heavily reliant on the quality and availability of data. Inaccurate or incomplete datasets can lead to flawed predictions, undermining research efforts. Thus, the need for high-quality, comprehensive datasets is crucial for AI to deliver reliable results.
2. Model Generalizability and Robustness
AI models must be robust and generalizable to handle the complexities of biological systems. Pharmaceutical research often involves diverse variables that impact drug efficacy, and AI models must be adaptable to different conditions and datasets. Ensuring that AI models work in real-world scenarios remains a challenge, requiring continuous updates and validation.
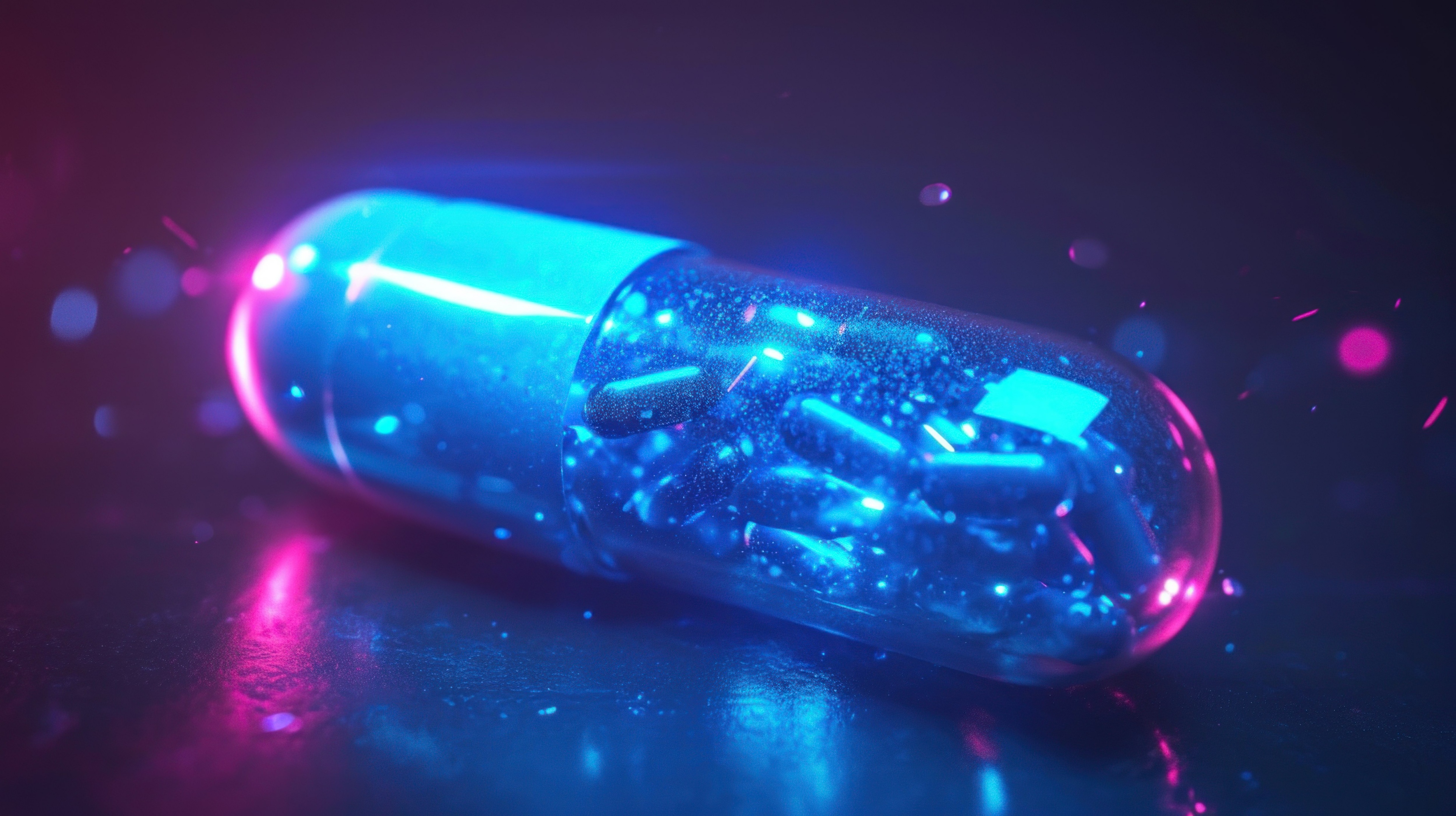
The Future of AI in Pharmaceutical Research
Despite the challenges, the future of AI in pharmaceutical research is full of promise. As AI models evolve and data availability improves, AI will continue to accelerate drug development and enhance research efficiency.
Personalized Medicine
One of the most exciting prospects for AI in pharmaceutical research is its ability to enable personalized medicine. By analyzing patient-specific data, AI can help design treatments tailored to individual patients, improving treatment efficacy and minimizing adverse effects. As AI technologies advance, personalized medicine will become a key focus in pharmaceutical research.
Increased Automation and Efficiency
As AI becomes more sophisticated, we can expect greater automation in pharmaceutical research, further reducing the time and cost associated with drug discovery. This will enable researchers to focus on innovation, developing novel therapeutics at an accelerated pace.
Expanding AI Applications
The applications of AI in pharmaceutical research are not limited to target identification and compound screening. AI technologies will be integrated into all stages of drug development, including clinical trial design, regulatory compliance, and post-market surveillance, enhancing the overall efficiency of the pharmaceutical industry.
Conclusion
AI is transforming pharmaceutical research, bringing significant advancements in drug discovery, development, and optimization. From identifying new drug targets and repurposing existing drugs to predicting toxicity and designing new molecules, AI is streamlining every aspect of the drug development process. Although challenges like data quality and model generalizability remain, the potential for AI to revolutionize pharmaceutical research is undeniable. As Vora et al. (2023) conclude:
The continued investment in and exploration of AI in the pharmaceutical industry offer exciting prospects for enhancing drug development processes and patient care.![]()
With the continuous evolution of AI technologies, the future of pharmaceutical research promises faster, more effective drug development, ultimately leading to better patient outcomes and more personalized therapies.
Read More in the Aimed Analytics Blog
Overcoming Common Challenges in Omics Data Analysis: How can deep learning unravel the complexities of vast omics data to drive medical innovation? Dive into the strategies that are turning data challenges into eye-opening and opportunity-generating insights in the biological sciences.
The Future of Genomics Data Analysis with AI: Discover how the integration of Artificial Intelligence is revolutionizing genomics, unlocking new possibilities in precision medicine, drug discovery, and early disease detection, while addressing challenges of data privacy and model transparency. Explore the future of healthcare innovation as AI and genomics converge to transform our understanding of human biology.
AI Revolution in Healthcare: 5 Breakthrough Uses: Discover how AI is reshaping the future of medicine with groundbreaking innovations in oncology imaging, ultrasound disease prediction, clinical trials, and more. From enhancing diagnostic accuracy to revolutionizing patient care, explore five amazing use cases where AI stands at the forefront of medical advancement.